ERC Consolidator Grant NEURAL SPICING: Neural Spectral Image Decoding
< Back to Research overview
Had we the capacity to image the molecular tissue composition in real time, without exposing the patient or clinical staff to radiation, then this would revolutionize healthcare. Spectral imaging techniques, such as multispectral diffuse reflectance imaging and photoacoustics, have the potential to recover important tissue properties including oxygenation, temperature and the concentration of water. However, decades of research invested in solving the inverse problem of reconstructing clinically relevant tissue properties from spectral measurements have failed to produce solutions that can quantify tissue parameters robustly in a clinical setting. Initial attempts to address the limitations of model-based approaches with machine learning were hampered by the absence of labeled reference data needed for supervised algorithm training. While training data simulation can potentially address this bottleneck, the domain gap between real and simulated images remains a huge unsolved challenge.
This project at the intersection of physics, biology, medicine, and data science bridges the domain gap with a "learning-to-simulate" approach and reframes the quantification problem as a decoding problem that can be tackled with physics-constrained neural networks. It is based on the hypothesis that unlabeled and weakly labeled measurement data can be leveraged to improve the realism of simulated spectral images and ultimately the quantification accuracy. In building upon modern machine learning techniques, the concept naturally allows different sources of uncertainty to be handled and even exploited, requires no labeled data for the target task, and enables imaging systems to learn from their experience.
The proposed second generation of spectral imaging is inherently safe and low-cost and could thus pave the way for a new era in interventional healthcare, with clinical applications ranging from cancer diagnosis to the staging and therapy of cardiovascular and inflammatory diseases.
Neural spectral image decodingfor quantitative optical imaging in interventional healthcare. A physics-based simulation framework for virtual spectral imagingcontinuously adapts itself to real data in order to minimize the domain gapbetween real and simulated data. A neural network-based decoding framework leverages both simulated data and real data to learn the reconstruction of relevant tissue properties from measurement data. As the project relies on the explicit and fast decomposition of all of the different parameters which are relevant for the image formation process (e.g. tissue temperature, oxygenation, concentration of water/lipids), it offers spectacular opportunities for a wide range of clinical applications in interventional healthcare.
© dkfz.de
Funding
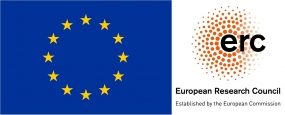
This project receives funding from the European Research Council (ERC) under the European Union's Horizon 2020 research and innovation programme (Grant agreement No. 101002198)