NCT Data Science Seminar
Das NCT Data Science Seminar ist eine campusweite Initiative, die führende Forscher im Bereich der Datenwissenschaft zusammenbringt, um methodische Fortschritte und medizinische Anwendungen zu diskutieren.
Um stets über kommende Vorträge informiert zu bleiben, abonnieren Sie unseren mailing list.
Upcoming & Recent Talks
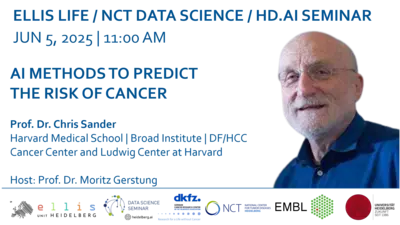
BioQuant, INF 267, Lecture Hall SR041
Bioskech
Chris Sander started his career as a theoretical physicist and then switched to theoretical biology, in part inspired by the first completely sequenced genome. He founded two departments of computational biology - at the EMBL in Heidelberg and Memorial Sloan Kettering Cancer Center in New York - and co-founded the research branch of the European Bioinformatics Institute in Cambridge and a biotech startup with Millennium in Boston.
Chris joined the Harvard community in 2016 as faculty in Cell Biology and then Systems Biology. Special Advisor for Quantitative Biology to the Ludwig Center at Harvard, and Associate Member of the Broad Institute. He is creating new connections between scientists at Dana-Farber and Harvard Medical School, including building translational collaborative bridges for scientists using quantitative sciences to solve biological problems.
With his group and collaborators, Chris aims to beat drug resistance in cancer using systems biology methods to develop combination therapies. They are also developing the next generation cBioPortal for cancer research and therapy, obtaining biomolecular structures and functional interactions on a large scale using evolutionary information, and adapting machine learning methods to mine millions of genomes. He is collaborating with groups in Denmark and the US to apply AI to longitudinal health records to identify patients at high risk for pancreatic and ovarian cancer and collaborates with clinicians in the design of effective affordable surveillance program aiming at the early detection of cancer.
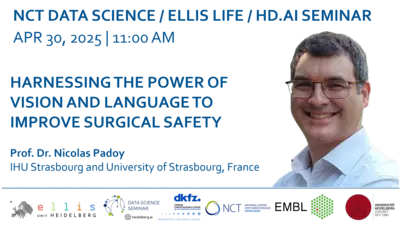
Abstract
Even though surgery has become safer and more efficient over the last several decades, preventable intra-operative adverse events still occur in a large number of cases. In this talk, I will present how intra-operative data in operating rooms (ORs) can be leveraged to detect, analyze, and support surgical activities. In particular, I will highlight how artificial intelligence can improve surgical quality and safety by providing clinicians and staff with cognitive aids that can enhance the performance of timeout and safety procedures, both within the OR by analyzing team activities and within the patient by analyzing tool tissue interactions. I will present recent unsupervised approaches aimed at analyzing video data captured from multiple room and endoscopic cameras and at scaling AI deployment in surgery by reducing dependency on annotations and expanding to a broader range of clinical applications. I will conclude with our current efforts to build a generalist vision-language model for surgery, trained using supervisory signals derived from surgical video lectures available on e-learning platforms, that can tackle in a zero-shot manner multiple surgical tasks and procedures without fine-tuning. By integrating all these approaches, we aim to build a surgical control tower for the OR of the future that is able to understand surgical processes and assist surgical teams, thus improving surgical care for patients.
Bio
Nicolas Padoy is a Professor of Computer Science at the University of Strasbourg, France, and the Scientific Director as well as Director of Computer Science and Artificial Intelligence Research at the IHU Strasbourg, a leading institute for minimally invasive surgery. He leads the CAMMA research group (Computational Analysis and Modeling of Medical Activities), which focuses on leveraging multimodal data from operating rooms with machine learning and computer vision to develop cognitive assistance systems and enhance human-machine collaboration. His research aims to improve the safety, quality, and efficiency of surgical procedures. In 2020, Nicolas Padoy was awarded a national AI Chair by the Agence Nationale de la Recherche (ANR) for his project AI4ORSafety and, in 2023, a prestigious European ERC Consolidator Grant for his project CompSURG. He was elected MICCAI Fellow in 2024 and is currently General Chair of the international IPCAI conference. Nicolas Padoy completed his PhD in 2010 jointly between the Technical University of Munich, Germany, and INRIA/University Henri Poincaré, France. Subsequently, he was a postdoctoral researcher and later an Assistant Research Professor in the Laboratory for Computational Interactions and Robotics at the Johns Hopkins University, USA.
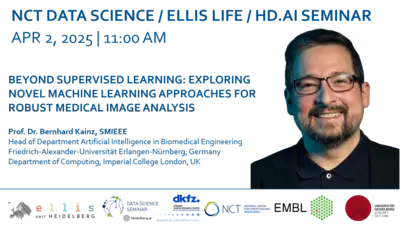
Abstract:
Machine learning has been widely regarded as a solution for diagnostic automation in medical image analysis, but there are still unsolved problems in robust modelling of normal appearance and identification of features pointing into the long tail of population data. In this talk, I will explore the fitness of machine learning for applications at the front line of care and high throughput population health screening, specifically in prenatal health screening with ultrasound and MRI, cardiac imaging, and bedside diagnosis of deep vein thrombosis. I will discuss the requirements for such applications and how quality control can be achieved through robust estimation of algorithmic uncertainties and automatic robust modelling of expected anatomical structures. I will also explore the potential for improving models through active learning and the accuracy of non-expert labelling workforces.
However, I will argue that supervised machine learning might not be fit for purpose, as it cannot handle the unknown and requires a lot of annotated examples from well-defined pathological appearance. This categorization paradigm cannot be deployed earlier in the diagnostic pathway or for health screening, where a growing number of potentially hundred-thousands of medically catalogued illnesses may be relevant for diagnosis.
Therefore, I introduce the idea of normative representation learning as a new machine learning paradigm for medical imaging. This paradigm can provide patient-specific computational tools for robust confirmation of normality, image quality control, health screening, and prevention of disease before onset. I will present novel deep learning approaches that can learn without manual labels from healthy patient data only. Our initial success with single class learning and self-supervised learning will be discussed, along with an outlook into the future with causal machine learning methods and the potential of advanced generative models.
Bio:
Bernhard Kainz is a full professor at Friedrich-Alexander-University Erlangen-Nuremberg where he heads the Image Data Exploration and Analysis Lab (www.idea.tf.fau.eu) and he is Professor for medical image computing in the Department of Computing at Imperial College London where he leads the human-in-the-loop computing group and co-leads the biomedical image analysis research group (biomedia.doc.ic.ac.uk). Bernhard's research is dedicated to developing novel image processing methods that augment human decision-making capabilities, with a focus on bridging the gaps between modern computing methods and clinical practice.
His current research questions include: Can we democratize rare healthcare expertise through Machine Learning, providing guidance in real-time applications and second reader expertise? Can we develop normative learning from large populations, integrating imaging, patient records and omics, leading to data analysis that mimics human decision making? Can we provide human interpretability of machine decision making to support the 'right for explanation' in healthcare?
Bernhard's scientific drive is documented with over 150 state-of-the-art-defining scientific publications in the field. He works as a scientific advisor for ThinkSono Ldt./GmbH., Ultromics Ldt., Cydar medical Ldt., as co-founder of Fraiya Ldt., and as a clinical imaging scientist at St. Thomas' Hospital London and has collaborated with numerous industries. He is an IEEE Senior Member, senior area editor for IEEE Transactions on Medical Imaging, and has won awards, prizes, and honours, including several best paper awards. In 2023, his research was awarded an ERC Consolidator grant.
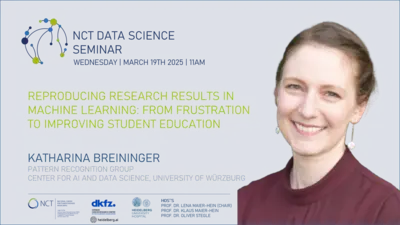
We have all been there: we read about an exciting new method in a paper, only to discover that the accompanying code is missing, incomplete, or nearly impossible to run—far from allowing us to reproduce the reported results. In the fast-paced world of looming computer science, machine learning, and computer vision conference deadlines, ensuring reproducibility often takes a back seat. This problem can also be seen or is even more pronounced for medical applications, where datasets are often not publicly available.
In this talk, I will share our experiences from a joint initiative between the University of Erlangen (Bernhard Egger and Andreas Kist) and the University of Würzburg (myself) to address this issue by integrating reproducibility into the curriculum for AI and computer science students. After first experiences with a dedicated Reproducibility Hackathon, we have subsequently established student projects for both Bachelor’s and Master’s students, focusing on reproducing results from published research papers. I will discuss the lessons we have learned, the challenges we have encountered, and our efforts to embed reproducibility as a core element of student education.
Bio:
Katharina Breininger leads the Pattern Recognition Group at the Center for AI and Data Science at the University of Würzburg. With her team, she develops labeling strategies and robust machine learning approaches for small-data settings in different interdisciplinary domains, with a focus on medicine and medical imaging.
After studying computer science in Marburg and Erlangen, she completed her PhD on image fusion during minimally invasive interventions at the Pattern Recognition Lab (Friedrich-Alexander-University Erlangen-Nürnberg) and Siemens Healthineers. Before joining the University of Würzburg in 2024, Katharina served as an assistant professor at FAU Erlangen-Nürnberg, leading the "Artificial Intelligence in Medical Imaging" group.
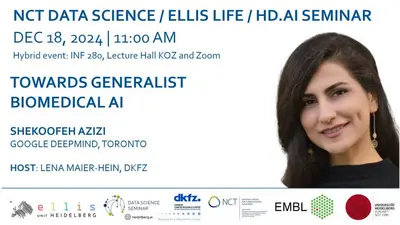
Foundation models have changed how we develop medical AI. These powerful models, trained on massive datasets using self-supervised learning, are adaptable to diverse medical tasks with minimal additional data and paved the way for the development of generalist medical AI systems. In this talk we will explore the capabilities of these models from medical image analysis, to polygenic risk scoring, and aiding in therapeutic development. Additionally, we will discuss the future of generalist and generative models in healthcare and science.
Bio:
Shekoofeh (Shek) Azizi is a staff research scientist and research lead at Google DeepMind, where she focuses on translating AI solutions into tangible clinical impact. She is particularly interested in designing foundation models and agents for biomedical applications and has led major efforts in this area. Shek is one of the research leads driving the ambitious development of Google's flagship medical AI models, including REMEDIES, Med-PaLM, Med-PaLM 2, Med-PaLM M, and Med-Gemini. Her work has been featured in various media outlets and recognized with multiple awards, including the Governor General's Academic Gold Medal for her contributions to improving diagnostic ultrasound.
Recorded Talks
Kontaktieren Sie uns
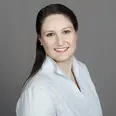