Intelligent Medical Systems
- Imaging and Radiooncology

Prof. Dr. Lena Maier-Hein
Head of Division, Managing Director "Data Science and Digital Oncology"
Artificial intelligence (AI) is set to revolutionize various areas of everyday life. In healthcare, however, its integration into routine procedures and impact on patients are still limited. The mission of the Division of Intelligent Medical Systems is to tackle the unique challenges of medical imaging AI to generate lasting patient benefit. A particular focus of our research lies in the surgical application domain.
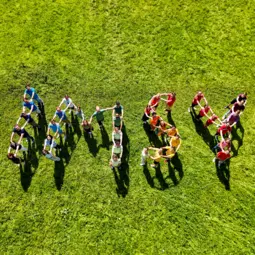
Our Research
Currently, patient outcome is heavily influenced by the surgical team's experience, with many potential complications being avoidable. To address such major socioeconomic problems and consistently elevate patient outcome beyond current standards, our multidisciplinary team works on intelligent medical systems in close collaboration with clinicians. Specific goals lie in the development of novel AI-enabled imaging techniques and trustworthy surgical AI systems that are ready for real-world clinical application. Key methodological challenges are related to the generalization of AI methods across devices, patient populations and hospitals, high data variability in an inherently sparse data regime, multimodal data integration, incorporation of prior knowledge into models, and the meaningful validation of AI systems under real-world clinical conditions.
Our Team Philosophy
Our division strongly believes in teamwork – not as an abstract ideal, but as a lived reality every day. Members of our division are involved in projects beside their own, making our group a strong network where everyone benefits from skills and talents that complement each other.
Our Team
-
Prof. Dr. Lena Maier-Hein
Head of Division, Managing Director "Data Science and Digital Oncology"
-
Dr. Alexander Seitel
Deputy Head of Division
-
Dr. Annika Reinke
Deputy Head of Division
-
Dr. Leonardo Ayala Menjivar
Group lead
-
Patrick Godau
Group lead
-
Alexander Baumann
Ph.D Student
-
Christoph Julien Bender
Ph.D Student
-
Dr. Evangelia Christodoulou
PostDoc
-
Kris Dreher
Ph.D Student
-
Prof. Dr. Alfred Franz
Scientist
-
Michaela Gelz
Administration and Technical Support
-
Pascal Hansen
Master Student
-
Dr. Doreen Heckmann-Nötzel
Scientific Manager
-
Jakob Hennighausen
Master Student
-
Niklas Holzwarth
Ph.D Student
-
Marco Hübner
Ph.D Student
-
Vera Klütz
Ph.D Student
-
Marcel Knopp
Ph.D Student
-
Nina Kraft
Administration and Technical Support
-
Ziying Li
Ph.D Student
-
Lucas Luttner
Ph.D Student
-
Leon Mayer
Ph.D Student
-
Dr. Keno März
-
Dominik Michael
Ph.D Student
-
Klavdiia Naumova
Ph.D Student
-
Jan-Hinrich Nölke
Ph.D Student
-
Piermarco Pascale
Computer Scientist for System Integration
-
Berit Pauls
Ph.D Student
-
Ahmad Qasim
Ph.D Student
-
Tim Rädsch
Ph.D Student
-
Maike Rees
Ph.D Student
-
Tom Rix
Ph.D Student
-
Stefanie Strzysch
Administration and Technical Support
-
Dr. Ömer Sümer
PostDoc
-
Dr. Minu Dietlinde Tizabi
PostDoc
-
Thuy Nuong Tran
Ph.D Student
-
Viet Tran Ba
Ph.D Student
Interested in joining IMSY?
Are you interested in our research and would like to carry out a student research project, bachelor, master, or diploma thesis? Or would you like to write your PhD thesis in our division?
News
Please refer to the following channels to get the latest news of our division:
Awards
We are proud of the significant recognition we have achieved through various awards. These awards demonstrate the impact of our work on the scientific community and beyond.
Media
Our engagement with the scientific community and the public is reflected in a diverse range of activities, including seminars, invited talks, conference presentations, and broad media coverage.
Teaching
The Division of Intelligent Medical Systems hosts the following seminars:
The Division of Intelligent Medical Systems offers the following university lectures:
Selected Publications
Godau P, Kalinowski P, et al.
Maier-Hein L, Reinke, A, Godau P et al.
Ayala L, Adler T, et al.
Maier-Hein L, et al.
Ardizzone L, …, Maier-Hein L, et al.
Get in touch with us
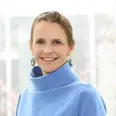