Automatic segmentation of bone lesions in multimodal image series
Motivation
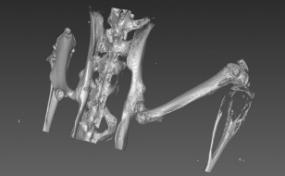
Figure 1 Rat skeleton with osteolytic lesion caused by bone metastases
© dkfz.de
Patients with malign bone lesions, for example bone metastases or multiple myeloma, suffer from pain, fractures and immobility. Imaging with CT and MRI allows the localization of these lesions. Functional parameters can be determined by dynamic contrast enhanced MRI (DCE MRI) or diffusion weighted MRI (DWI).
To quantify the bone lesions and the tumor mass the volume of the lesions is determined based on morphological imaging. For the volumetry of the lesions an accurate segmentation is needed. Manual segmentation of bone metastases and bone lesions in multiple myeloma is a very time consuming task and cannot be reproduced. In multiple myeloma a manual segmentation of all lesions is not possible because of the large number of lesions and the huge amount of image data in multiple modalities. Without automating of the segmentation and image analysis process the determination of the overall tumor mass is not feasible. With computerized tools that detect bone lesions in the image data automatically the analysis of all lesions is possible, even for large data sets in clinical trials.
Bone metastases in rat model

Figure 2 Soft tissue tumor in right hind leg in T2 MRI and parameter A (permeability) in DCE MRI
© dkfz.de
The development of segmentation methods for bone lesions is at first performed on preclinical data under controlled conditions. In this project bone metastases in case of mamma carcinoma in rats are analyzed. Bone metastases show a soft tissue tumor part and changes in bone structure. The bone structure changes occur as bone resorption (osteolysis) or bone formation (osteoblastic lesion).
For the rat model tools are developed that segment automatically the bone lesion and the soft tissue tumor. With dynamic imaging techniques (dynamic contrast enhanced MRI – DCE MRI, diffusion weighted imaging – DWI) parameters for blood volume, vessel permeability and cell density can be determined. These functional parameters shall be included in an automatic classification of tumor tissue regions in active or necrotic tumor tissue.
Bone lesions in patients with multiple myeloma

Figure 3 Multiple myeloma patient with multiple lesions.
© dkfz.de
Multiple myeloma is a malign hematological disease that shows similar effects on bone as in bone metastases. Bone lesions can occur as delimited focal lesions or as diffuse osteoporosis. With whole body MRI and CT these lesions can be detected but the large amount of image data and the multitude of lesions makes an exact quantitative analysis of all lesions impossible. Determination of the overall tumor mass requires an exact segmentation of all lesions. A manual segmentation of all lesions is too complex and therefore not viable. For a follow-up analysis currently only a reference lesion is selected and quantified. Without computerized tools that perform an automatic segmentation the quantification of the overall tumor mass is not possible.
Here automatic segmentation methods for the delineation of bone lesions in multiple myeloma are developed to enable the quantification of the overall tumor mass.
Functional parameters from DCE-MRI and DWI are used for the analysis of a therapy effect but also for a distinction between malign and benign tissue changes. Additional to the morphological information from CT and MRI these dynamic parameters shall be integrated in the automatic analysis of bone lesions in multiple myeloma.
Cooperation
Animal model
PD Dr. med. Tobias Bäuerle, group leader „Research Group Molecular Imaging“, Division of Medical Physics in Radiology
Multiple Myeloma
Dr. med. Jens Hillengass, group leader „Imaging in Multiple Myeloma“, Heidelberg University Hospital
Segmentation tools
Prof. Dr. H.P. Meinzer, head of the Department “Division of Medical and Biological Informatics”
Publications
Fränzle A; Hillengaß J; Bendl, R: Spinal focal lesion detection in multiple myeloma using multimodal image features. In: Proc. SPIE 9414, Medical Imaging 2015: Computer-Aided Diagnosis. Hrsg.: L. M. Hadjiiski, G. D. Tourassi. 94143B
Sauer S, Fischer AM, Fraenzle A, Kunz C, Merz M, Wuchter P, Schmaus M, Ho AD, Goldschmidt H, Hillengass J: Impact of Radiation Therapy on Stem Cell Harvest in Multiple Myeloma. Blood, 124 (21) (2014).
Fränzle A: Strategien zur Quantifizierung von osteolytischen Läsionen bei ossären Tumorerkrankungen. Dissertation Medizinische Fakultät, Universität Heidelberg (2014).
Fränzle A; Sumkauskaite M; Hillengass J; Bäuerle T; Bendl R: Fully automated shape model positioning for bone segmentation in whole-body CT scans. Journal of Physics / Conference Series, 489 (2014) Art. Nr. 012029
Bretschi M; Fränzle A; Merz M; Hillengass J; Semmler W; Bendl R; Bäuerle T: Assessing Treatment Response of Osteolytic Lesions by Manual Volumetry, Automatic Segmentation, and RECIST in Experimental Bone Metastases. Academic Radiology, 21 (2014) 1177-1184
Fränzle A; Bretschi M; Bäuerle T; Giske K; Hillengass J; Bendl R: A quantification strategy for missing bone mass in case of osteolytic bone lesions. Medical Physics, 40 (12) (2013) Art. Nr. 123501.
Fränzle A; Bretschi M; Bäuerle T; Giske K; Hillengass J; Bendl R: Quantification of osteolytic bone lesions in a preclinical rat trial. In: AIP Conference Proceedings 1559 of the International Symposium on Computational Models for Life Sciences. Hrsg.: C. Sun, T. Bednarz, T.D. Pham, P. Vallotton, D.Wang. AIP Publishing, (2013) 307-312.
Fränzle A; Bretschi M; Bäuerle T; Bendl R: Automatic Detection of Osteolytic Lesions in Rat Femur With Bone Metastases. In: Bildverarbeitung für die Medizin 2013. Algorithmen – Systeme – Anwendungen. Hrsg.: H.-P. Meinzer, T.M. Deserno, H. Handels, T. Tolxdorff. Springer Verlag Berlin Heidelberg New York, (2013) 314-319.
Fränzle A; Bendl R: Automatische Segmentierung und Klassifizierung von Knochenmarkhöhlen für die Positionierung von Formmodellen. In: Bildverarbeitung für die Medizin 2012. Algorithmen – Systeme – Anwendungen. Hrsg.: T. Tolxdorff, T.M. Deserno, H. Handels, H.-P. Meinzer,. Springer Verlag Berlin Heidelberg New York, (2012) 280-284.
Fraenzle, A., Bendl, R.: Fully automated model positioning for shape-based bone segmentation. Radiotherapy and Oncology 99 (Suppl.1), (2011) S480 (Poster).