Biostatistics
- Cancer Risk Factors and Prevention

Prof. Dr. Annette Kopp-Schneider
Head
The Division of Biostatistics‘ mission is to support scientists in performing and publishing excellent reproducible research. We develop efficient experimental designs and devise sound statistical analysis and interpretation of biomedical data. Adequate statistical methods are rarely available ‘off the shelf’ but must be developed and tailored to the specific problem in collaboration with the biomedical researcher. Hence, the Biostatistics group acts as research division with service function.
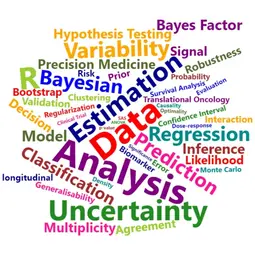
Our Research
The mission of the Division of Biostatistics is to support DKFZ scientists in performing and publishing excellent reproducible research. Biostatistics is an interdisciplinary science with the aim to provide efficient design of experiments and trials, and devise sound statistical analysis and interpretation of biomedical data. Adequate experimental design and analysis strategies are rarely available ‘off the shelf’ but must be developed and tailored to the specific problem in collaboration with the biomedical researcher. Therefore, the Division of Biostatistics can only provide state-of-the-art support if it actively performs methodological research and implements newly developed analysis strategies. As a consequence, it acts as a research division with a service function.
Our methodological research activities cover a wide range of biostatistical topics, often motivated and interlinked with long-standing collaborations within and outside the DKFZ, including a large number of clinical trials. The close collaboration with biomedical researchers and clinicians allows us to link statistical methodological research and clinical practice, thus contributing to the advancement of translational oncology and precision oncology. Major areas of current research interest include: design and analysis of clinical trials, both in the frequentist setting as well as in the Bayesian framework; identification of prognostic and particularly predictive factors from clinical and molecular data; optimal design and analysis for dose-response relationships, with a focus on combination of substances; measuring dependence between sets of random variables for various data types. We are keen on approaching novel methodological challenges, and indeed, in our collaborations with biomedical scientists, we address a variety of additional research topics. More detailed information about our research activities are given here.
The working group “Statistics in translational research” within the Division of Biostatistics supports clinical trial groups as biometric center and bridges research on molecular patient characteristics to new therapeutic options in oncology.
Biostatistical Service and Support
We provide statistical support for all scientific activities at the DKFZ, from in vitro and animal to human subject studies. Our support covers experimental design, sample size/power estimation, data analysis, software guidance, visualization and interpretation of statistical results, and preparation of results for publication. It ranges from brief statistical consultations to long-term collaborations and covers standard statistical analysis approaches as well as the development of complex statistical methods tailored to specific questions. We offer discussions on advantages and disadvantages of different statistical methods and guidance for the method of choice in specific cases.We provide assistance on statistical aspects and requirements of funding applications, ethical vote applications, clinical trial protocols and animal studies.
For standard experiments (no high-throughput measurements) recorded in spreadsheet files, samples/observations/replicates should be entered in rows, features/characteristics in columns. If multiple measurements per sample have been made (e.g. time series), each measurement should go into a separate row and an identifier variable for samples should be included. Column names should not contain any special signs. If measurements are coded, a legend must be provided. Dates should all be in the same format. If during the process of analysis your data must be updated or corrected, please provide an updated file without changing column names, formats etc. Information supplied by highlighting, coloring or any other type of formatting cannot be imported and used for the analysis.
The DKFZ provides SPSS SigmaPlot for standard analysis in a user-friendly environment. GraphPad Prism is another user-friendly statistical software frequently used at the DKFZ but without a campus-wide license. The Genomics and Proteomics Core Facility provides bioinformatics tools for conducting standard microarray/sequencing analysis, such as Chipster and IPA. Our division generally uses R/Bioconductor and SAS for power/sample- size estimations.
We consider reproducible research to be essential for scientific work. For this reason, we prepare our analysis in R/Bioconductor in combination with Sweave/Knitr in order to allow for reproducibility of results, figures and tables. If requested, we can also provide stand-alone analysis scripts that can be used to reproduce results and can be submitted along with your manuscript.
We encourage PhD candidates and their supervisors to contact us whenever they need statistical advice on their experimental design, the methods to use, the correct application of statistical software, or the proper interpretation of results. We normally expect PhD candidates to perform the statistical analyses for their theses themselves. Of course, in case of a more complex analysis requiring advanced statistical knowledge and/or software expertise we will provide the necessary support.
Please email the division of Biostatistics at biostatistics-consulting(at)dkfz.de and briefly describe your experiment/question and your aim.
Statistics Courses
The division of Biostatistics offers three consecutive statistics lecture series starting every summer semester.The aim of the courses is to enable the participants to perform simple analyses by themselves, to recognize when professional statistical advice is needed and to facilitate cooperation between researchers and the division of Biostatistics. The topics that are covered are chosen according to the needs of researchers at the DKFZ. For details about dates and location please visit the Training Portal (for DKFZ employees on the intranet), the Heidelberg University Lecture Index, or contact the division of Biostatistics.
Lecture series for researchers and PhD students in the biological or clinical sciences without prior knowledge in statistics.
Topics:
- Descriptive statistics: plots, measures of location and spread
- Confidence intervals
- Statistical hypothesis testing, p-value, etc.
- Statistical tests for quantitative data, e.g., t-test
- Statistical tests for qualitative data, e.g., chi-square test
- Correlation and regression
- Study design
This lecture series accompanies "Basic Principles of Biostatistics" and shows how the methods introduced there are coded in R. Participants should have a working R installation on their computers. Also, participants should be familiar with the statistical concepts covered in the "Basic Principles in Biostatistics" lecture.
Team-taught lecture series by members of the division of Biostatistics for researchers and PhD students in the biological or clinical sciences with basic knowledge of statistics.
Topics:
- Analysis of Variance
- Non-parametric methods
- Multiple linear regression
- Logistic regression
- Linear mixed models
- Dose-response modeling
- Diagnostic tests
- Measuring agreement
- Survival analysis: Kaplan-Meier curves, logrank tests, Cox PH regression
- Variable selection in regression
- Design of clinical trials
- Multiple Testing
- Introduction to Bayesian thinking
This lecture series accompanies "Advanced Topics in Biostatistics" and shows how the methods introduced there are coded in R. Participants should have some basic R programming skills, including the ability to use the basic statistical methods shown in the "Basic principles" course.
In addition to the courses organized by the division of Biostatistics, the Advanced Training department of the DKFZ also offers programming courses in R and SAS, and the Genomics and Proteomics Core Facility at DKFZ offers courses on specific data analysis tools for high-throughput genomics data. DKFZ employees please visit the Training Portal for further information.
Research Topics
The Division of Biostatistics currently focuses on several research topics:
This research area deals with innovative methods for clinical trial designs and evaluation strategies for clinical data. Motivated by our involvement in a multitude of clinical trials in all phases, we develop methods for design and analysis of clinical trials, both in the frequentist setting as well as in the Bayesian framework.
One focus in oncology lies in the prediction of clinical endpoints from a set of candidate clinical and biological covariates to support evidence for individualized treatment recommendations. We extend standard prediction methods to include high-dimensional covariate data, and we investigate their prediction performance. We focus on time-to-event endpoints and also consider competing risks settings and multi-state modeling.
As dose/concentration-response experiments are commonly performed at the DKFZ, we develop optimal design and analysis procedures for dose-response relationships, especially when combinations of substances are investigated. Dose-response experiments are particularly valuable to identify susceptibility of individual tumor samples to specific available drugs.
Distance correlation is a powerful measure of dependence between random variables. We develop distance correlation methods for biomedical data including time-to-event settings and genomic measurements.
We are in intense collaboration with a number of groups and provide essential contributions by developing methodology for the emerging scientific questions, thus making the collaboration a statistical research field. A selection of these research projects is described here.
Software
Bayesian design for phase II trials
The WebApp BDP2 provides a workflow to determine design parameters for a multi-stage single-arm phase II trial with binary endpoint. Declaration of efficacy and futility is based on the Bayesian posterior distribution. It is based on the R-package BDP2 available from CRAN.
For details see:
Kopp‐Schneider, A., Wiesenfarth, M., Witt, R., Edelmann, D., Witt, O., & Abel, U. (2019). Monitoring futility and efficacy in phase II trials with Bayesian posterior distributions - A calibration approach. Biometrical Journal, 61(3), 488-502.
Sample size calculation for modifications of Simon's two-stage design
The R package hctrial can be used to calculate the sample size for modifications of Simon's two stage design allowing for stratification and incorporation of historical controls.
For details see:
Edelmann, D., Habermehl, C., Schlenk, R. F., & Benner, A. (2020). Adjusting Simon's optimal two‐stage design for heterogeneous populations based on stratification or using historical controls. Biometrical Journal, 62(2), 311-329.
Sample size determination for diagnostic studies
The WebApp SampleSizeDiagnosticTest can be used to estimate the sample size for a study where the aim is to test whether the performance of a diagnostic test is sufficient in terms of false positive (specificity) and true positive fraction (sensitivity).
mfp: Multivariable Fractional Polynomials
The mfp package is a collection of R functions targeted at the use of fractional polynomials (FP) for nonlinear modelling the influence of continuous covariates on the outcome in regression models. For details see:
Benner A. mfp - multivariable fractional polynomials. R News 2005; 5: 20-23
The EASIX calculator
A web implementation of the general mortality prediction model based on the Endothelial Activation and Stress Index (EASIX).
- Luft T. et al. EASIX in patients with acute graft-versus-host disease: a retrospective cohort analysis. Lancet Haematol. 2017;4(9):e414-e423. doi: 10.1016/S2352-3026(17)30108-4.
- Merz A. et al. EASIX for prediction of survival in lower-risk myelodysplastic syndromes. Blood Cancer J. 2019;9(11):85. doi: 10.1038/s41408-019-0247-z.
- Luft T. et al. EASIX and mortality after allogeneic stem cell transplantation. Bone Marrow Transplant. 2020;55(3):553-561. doi: 10.1038/s41409-019-0703-1.
- Jiang S. et al. Predicting sinusoidal obstruction syndrome after allogeneic stem cell transplantation with the EASIX biomarker panel. Haematologica. 2021;106(2): 446-453. doi: 10.3324/haematol.2019.238790.
- Luft T. et al. EASIX-1year and late mortality after allogeneic stem cell transplantation (Blood Adv., under review).
Extended inference for lasso and elastic-net regularized Cox and generalized linear models
The c060 package extends the popular R-package glmnet and provides additional functions particularly useful for high-dimensional risk prediction modelling, e.g. stability selection, estimation of prediction error (curves) and an efficient interval search algorithm for finding the optimal elastic net tuning parameter combination. Most functions offer improved computational efficiency through code parallelization.
For details see:
Sill M, Hielscher T, Becker N, Zucknick M (2014). C060: Extended Inference with Lasso and Elastic-Net Regularized Cox and Generalized Linear Models. Journal of Statistical Software 62(5) 1-22. www.jstatsoft.org/v62/i05/
Design of dose-response studies
The WebApp DoseResponseDesigns calculates optimal experimental designs for log-logistic and Weibull functions, including designs for combination experiments of two substances in a ray design. It also provides the D-efficiency of any given design compared to the optimal design.
For details see:
Holland-Letz, T; Kopp-Schneider, A: An R-shiny application to calculate optimal designs for single substance and interaction trials in dose response experiments. Toxicology Letters 337, 18-27. doi.org/10.1016/j.toxlet.2020.11.018
Analysis of dose-response studies
The WebApp MDRA performs dose-response analysis of multiple experiments. It allows for uploading of a csv-formatted data file for analysis. The four-parameter log-logistic model is used to fit dose-response data. Dose-response designs and data are visualized. Single experiments can be excluded from global analysis. Meta-analysis is used to average, e.g., EC50.
For details see:
Jiang, X, and Kopp-Schneider A. (2015). Statistical strategies for averaging EC50 from multiple dose-response experiments. Archives of Toxicology 89(11) 2119-2127. DOI 10.1007/s00204-014-1350-3
Jiang, X and Kopp-Schneider A. (2014). Summarizing EC50 estimates from multiple dose-response experiments: A comparison of a meta-analysis strategy to a mixed-effects model approach. Biometrical Journal 56(3): 493-512. DOI 10.1002/bimj.201300123
The dcortools package features a very efficient and flexible implementation of distance correlation allowing to calculate numerous generalisations and modifications. Distance correlation methods for survival are also included.
Open-source toolkit for analyzing and visualizing challenge results
ChallengeR is an R package for analyzing and visualizing challenge results in the field of biomedical image analysis and beyond intuitive way to gain important insights into the relative and absolute performance of algorithms.
For details see:
Wiesenfarth M, Reinke A, Landman BA, Eisenmann M, Saiz LA, Cardoso MJ, Maier-Hein L, Kopp-Schneider A. Methods and open-source toolkit for analyzing and visualizing challenge results. Sci Rep. 2021 Jan 27;11(1):2369. Doi: 10.1038/s41598-021-82017-6.
Cochran-Armitage Test for trend
The WebApp CATrend computes the one-sided p-values of the Cochran-Armitage trend test for the asymptotic and the exact conditional test. The Cochran-Armitage Test for trend is used in the analysis of 2 x k contingency tables with k ordered categories. It compares the null hypothesis of equal proportions in all k categories to the alternative of ordered proportions. Details, also about numerical calculation can be found in the WebApp. A corresponding R package (CATTexact) is available.
Clustering and visualization of mixed-type data
CluMix is an R package that provides utilities for clustering subjects and variables with mixed data types. The main feature is the creation of a mixed-data heatmap.
For details see:
Hummel M, Edelmann D, Kopp-Schneider A. Clustering of samples and variables with mixed-type data. PLoS One. 2017 Nov 28;12(11):e0188274. doi: 10.1371/journal.pone.0188274. eCollection 2017.
Visual analytics for the integrated analysis of microarray data
SEURAT provides interactive visualization capability for the integrated analysis of high-dimensional gene expression data. Gene expression data can be analyzed together with associated clinical data, array CGH (comparative genomic hybridization), SNP array (single nucleotide polymorphism) data and available gene annotations in an integrated manner.
For details see:
Gribov A*, Sill M*, Lück S, Rücker F, Döhner K, Bullinger L, Benner A, Unwin A (2010). SEURAT: visual analytics for the integrated analysis of microarray data. BMC Med Genomics;3:21. (* joint first authors). DOI: 10.1186/1755-8794-3-21
Biclustering via sparse singular value decomposition incorporating stability selection
s4vd is an addon package for the R-package biclust and provides implementations of the ssvd and s4vd algorithm to perform biclustering via sparse singular value decomposition with and without stability selection.
For details see:
Sill M, Kaiser S, Benner A and Kopp-Schneider A (2011). Robust biclustering by sparse singular value decomposition incorporating stability selection. Bioinformatics 27(15) 2089-2097. DOI:10.1093/bioinformatics/btr322
Working group "Statistics for Translational Oncology"
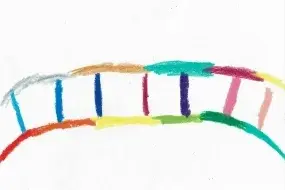
The working group contributes to bridging from research on molecular data to new therapeutic options for cancer patients ("The Bridge", painted by Deborah Kunz, 7 years)
One main focus is the exploitation of high-dimensional molecular data to improve the understanding of carcinogenesis and prediction of disease progression and treatment outcome. In the era of precision medicine, another area of focus is the search for prognostic biomarkers associated with disease progression and treatment outcome and for predictive genetic and genomic factors, i.e. the identification of biologically defined patient subgroups, who benefit from specific treatment or who are susceptible to serious adverse events due to their genomic profile. Another research topic is the development and validation of statistical methods for classification, prognosis and prediction using high-dimensional data. Further, we evolve data-driven model selection strategies in the framework of more complex multi-state models incorporating molecular data to capture pathogenic disease processes and underlying etiologies more precisely.
In addition to our methodological research, we also contribute to transferring research results from experimental and observational data into clinical practice. We collaborate on clinical trials and other forms of clinical research to convert the knowledge gained in the basic research into effective clinical applications.
For example, we support several trials of the NCT Precision Medicine in Oncology (PMO) program which has been established at the NCT Heidelberg. One example is the NCT-PMO-1602 phase II study CRAFT - Continuous ReAssessment With Flexible ExTension in Rare Malignancies.
We participate in the high-dimensional data topic group of the STRATOS (STRengthening Analytical Thinking for Observational Studies) initiative [Sauerbrei et al. 2014]. The main goal of STRATOS is to provide guidance for the design and analysis of studies with observational data.
Furthermore, we are involved in the HARMONY alliance, a European public-private partnership in hematology including hospitals, research institutes, patient organizations, pharmaceutical and IT companies. The primary aim of the alliance is to use big data to improve outcomes for patients with blood cancers.
For a broader overview of the projects we are or have been involved in, have a look at some of our long-term collaborations.
Collaborations
We collaborate with many researchers within and outside of DKFZ. We provide support for experimental design and perform statistical analyses tailored to the specific scientific question. Examples of major collaborations are:
The German-speaking Myeloma-Multicenter Group (GMMG) conducts active research to improve treatment methods for multiple myeloma. Therein our long-term collaboration with the GMMG has witnessed treatment modifications that have been/will be implemented in the German health system. So, the current standard of care for patients with newly diagnosed multiple myeloma includes chemo-combination therapy followed by autologous stem cell transplantation. Based on the analysis of the GMMG-MM5 trial, it was shown that patients aged 65 to 70 years benefit from stem cell transplantation in the same way as the age group <= 65 without additional safety risks [Mai EK, Miah K et al. 2021]. As a consequence, cost absorption of ASCT is now admissible for multiple myeloma patients up to the age of 70 years by statutory health insurances (cf. https://gmmg.info/atp/). In addition, in part 1 of the randomized phase III study GMMG-HD7, it could be shown that the addition of a novel immunotherapy client significantly reduces the risk of detecting residual disease in the bone marrow which is a surrogate for prolongation of progression-free survival. Based on the results of this IIT trial, the monoclonal antibody will be sought for regulatory approval [Goldschmidt et al 2022]. Furthermore, the test for free light chains in the blood has so far been an easy-to-use diagnostic tool for predicting tumor activity. It has now been shown that the normalization of free light chains has a prognostic impact on progression-free survival, allowing an individualized therapy for this subgroup of responding patients. [Klein EM, Tichy D et al., 2021]
The German-Austrian AML Study Group (AMLSG) is one of the world's largest study groups for the research and treatment of AML, initiating a number of innovative national and interventional clinical trials and running the AMLSG BiO Registry Study with around 1,500 newly diagnosed AML patients being recruited annually. All patients included in the AMLSG BiO Registry Study agree to a systematic central biobanking and undergo in-depth molecular and genetic diagnostics which allow for prestigious translational research projects that are published in high-impact journals. Members of the working group have been responsible statisticians in the clinical trials since the study group was founded in 2003 and support many of the accompanying research projects.
In cooperation with the Section of Allogeneic Stem Cell Transplantation at Heidelberg University Hospital we investigate the usefulness of EASIX as prognostic and predictive biomarker for several diseases and endpoints. For instance, we illustrate the prognostic and predictive value of EASIX for time-to-sepsis, the effectiveness of statin-based prophylaxis for non-relapse mortality in different EASIX subgroups and the prognostic value of EASIX for severe complications after CAR-T cell therapy.
- Goldschmidt et al. Addition of isatuximab to lenalidomide, bortezomib, and dexamethasone as induction therapy for newly diagnosed, transplantation-eligible patients with multiple myeloma (GMMG-HD7): part 1 of an open-label, multicentre, randomised, active-controlled, phase 3 trial. Lancet Hematology 9(11):e810-821 (2022). doi: 10.1016/S2352-3026(22)00263-0
- Klein EM, Tichy D et al. Prognostic Impact of Serum Free Light Chain Ratio Normalization in Patients with Multiple Myeloma Treated within the GMMG-MM5 Trial. Cancers 13(9): 4856 (2021). DOI: 10.3390/cancers13194856
- Mai EK, Miah K. et al. Bortezomib-based induction, high-dose melphalan and lenalidomide maintenance in myeloma up to 70 years of age. Leukemia 35(12): 3636 (2021). doi: 10.1038/s41375-021-01357-4.
- Sauerbrei W, et al. STRengthening analytical thinking for observational studies: the STRATOS initiative. Stat Med. 33(30):5413-5432 (2014)
- Goldschmidt et al. Addition of isatuximab to lenalidomide, bortezomib, and dexamethasone as induction therapy for newly diagnosed, transplantation-eligible patients with multiple myeloma (GMMG-HD7): part 1 of an open-label, multicentre, randomised, active-controlled, phase 3 trial. Lancet Hematology 9(11):e810-821 (2022). doi: 10.1016/S2352-3026(22)00263-0
- Klein EM, Tichy D et al. Prognostic Impact of Serum Free Light Chain Ratio Normalization in Patients with Multiple Myeloma Treated within the GMMG-MM5 Trial. Cancers 13(9): 4856 (2021). DOI: 10.3390/cancers13194856
- Mai EK, Miah K. et al. Bortezomib-based induction, high-dose melphalan and lenalidomide maintenance in myeloma up to 70 years of age. Leukemia 35(12): 3636 (2021). doi: 10.1038/s41375-021-01357-4.
- Sauerbrei W, et al. STRengthening analytical thinking for observational studies: the STRATOS initiative. Stat Med. 33(30):5413-5432 (2014)
Team
The task of the Biostatistics Department is to support scientists at the DKFZ in conducting and publishing excellent reproducible research.
-
Prof. Dr. Annette Kopp-Schneider
Head
-
Axel Benner
-
Dr. Silvia Calderazzo
-
Dr. Dominic Edelmann
-
Charlotte Eidloth
-
Johannes Epe
-
Varun Raj Ginde
-
Katrin Hakenesch
-
Thomas Hielscher
-
Prof. Dr. Tim Holland-Letz
-
Dr. Julia Krzykalla
-
Kaya Miah
-
Dr. Marilena Müller
-
Dr. Maral Saadati
-
Vivienn Weru
Selected publications
All Publications
Get in touch with us
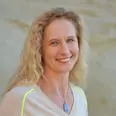