Systems Biology of Signal Transduction
- Functional and Structural Genomics

Prof. Dr. Ursula Klingmüller
Division Leader
Bridging from the cellular to the organ scale, the division aims at unraveling molecular mechanisms regulating cell communication and cell decisions, as a failure of these control mechanisms contributes to tumor development.
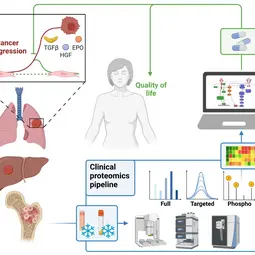
Our Research
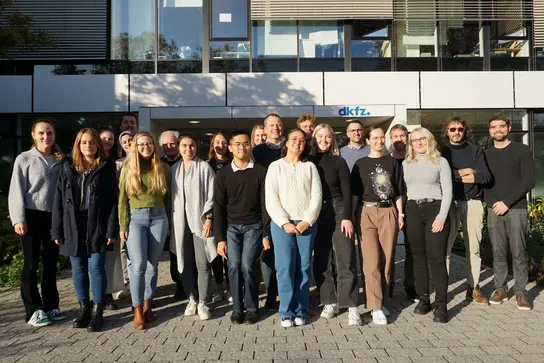
We combine quantitative technologies with mechanism-based mathematical modeling to enable early detection and personalized treatment of cancer to prevent tumor progression and improve quality of life.
Cellular responses are regulated by a multitude of extracellular signals received by cell surface receptors. Within cells the information is processed through complex intracellular signaling networks that in turn impinge on gene regulation. The dynamics of signal transduction is affected by the metabolic state of cells as well as the impact of signal transduction on metabolism. These multitude of reactions are integrated and converted to phenotypic responses such as proliferation, survival and differentiation. The majority of these responses are non-linear and operate on very different time scales, ranging from minutes to hours and days. Due to this complexity, it is of advantage to employ mathematical modelling approaches. Data-based mathematical models not only enable rapid testing of hypotheses to uncover deregulation in cancer, but also facilitate the prediction of trajectories such as disease development and support the establishment optimized intervention strategies to change the course of a disease.
In close cooperation with our mathematical modelling partners and the clinical partners, we are advancing cellular model systems to include an authentic extracellular matrix, developing cutting edge mass spectrometry approaches for clinical proteomics and increasingly combine AI-based modelling approaches with dynamic pathway modeling. The key areas of medical interest are processes determining the development of leukemia, lung cancer or liver cancer.
Areas of focus in the division are:
- Advancing systems medicine approaches for clinical translation through standardization of workflows for high-quality data generation and the development of predictive mathematical models
- Bridging from the single cell to the cell population and organ level to unravel principal mechanisms controlling erythropoietin (Epo)-induced cellular responses in the hematopoietic system.
- Resolving interactions in the tumor microenvironment determining lung cancer development and personalized optimization of intervention strategies.
- Deciphering the interconnection of signal transduction and metabolism to unravel mechanisms controlling the compensation of liver injury due to drugs or viral infection and to establish model-based biomarkers for early detection of liver cancer.
Outlook
Our ambition is to establish mathematical models for the prediction of disease trajectories to support early detection of cancer and to establish effective intervention strategies for converting an aggressively progressing cancer to a controllable disease. Therefore, we continue to develop integrative models linking signal transduction, metabolism and phenotypic responses that are essential building blocks for the establishment of mechanistic multi-scale models to predict the impact of cellular alterations on the entire organ. Our mass spectrometry based clinical proteomics pipeline greatly increases the possibilities to generate reliable quantitative data and through the combination of AI-based pattern recognition with dynamic pathway modeling much extends the development of predictive models. Through our clinical partners we have access to high-quality patient samples that allow to adapt our predictive mathematical models to disease states and to resolve mechanisms leading from chronic diseases to cancer and impacting the heart.
Current Research Interests
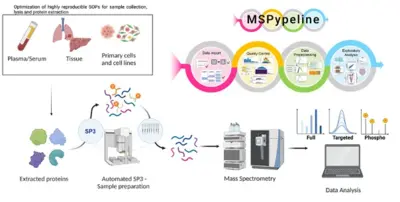
Advancement of clinical proteomics for systems medicine
Disease initiation and progression is a highly dynamic process and to pinpoint alterations resulting in deregulation, systems medicine approaches combining high-quality data generation with the development of mechanistic mathematical models are required. As biological functionality is executed by proteins and their abundance critically determine cell context specific information processing, a quantitative proteomics approach suitable for the generation of quantitative data for mathematical modelling and sufficiently robust for clinical translation is required. A critical basis for the development of a robust clinically-applicable pipeline is the collection of patient samples according to standard operating procedures (SOPs) (Wessels et al., Transl Lung Cancer Research 2020; Gegner et al., Front Mol Biosci 2022). Key steps of our clinical proteomics pipeline have been the optimization of the lysis of cells, extracellular matrix, tissue and blood plasma, semi-automated sample processing and the inclusion of quality standards. To ensure standardized data processing and thereby secure reproducibility of results the MSPypeline tool (Heming et al., Bioinform Adv 2022) was developed. With these developments in place, we could already demonstrate that the generated data much improves calibration of our mechanism-based dynamic pathway models that we are developing for TGFβ, HGF, EGF, IL-6, TNFα, IFNa and Epo-signal transduction.
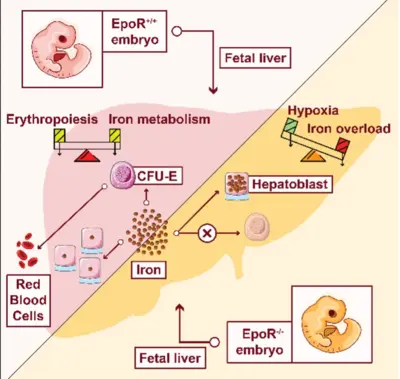
Bridging from the single cell to the cell population – Learning from mechanisms controlling erythropoietin (Epo)-induced cellular responses and erythroleukemia
In a healthy human individual, every second 2.5 million red blood cells (erythrocytes) are generated. Despite this high-proliferative capacity, erythroleukemia is rather rare. Key regulator of erythropoiesis is the hormone erythropoietin (Epo) that is secreted by the kidney and acts primarily on erythroid progenitor cells at the colony-forming unit-erythroid (CFU-E) stage. CFU-E cells express the Epo receptor (EpoR) and Epo is essential for their survival, proliferation and differentiation. Since CFU-E cells are readily accessible and can be cultivated in vitro, this system is highly suitable to establish strategies for high-quality data generation and to advance mathematical modeling approaches for resolving key regulatory mechanisms. Whereas at the cell population level, a graded increase in survival of CFU-E cells in response to Epo stimulation is observed, at the single cell level survival it is a binary decision. By a mathematical modelling approach, we resolved that heterogeneity in Epo induced STAT5 phosphorylation is due to variability in membrane associated processes and the cytoplasmic volume and defines a survival threshold. Thus, the cytoplasmic volume of CFU-E cells converts a switch-like behavior in individual cells to graded population level responses (Adlung et al., Cell Rep 2021). In the absence of the EpoR the production of hemoglobin in CFU-E cells fails and a massive iron overload is observed in the fetal liver of EpoR knockout embryos, which is accompanied by a reduction in iron-sulfur cluster proteins involved in oxidative phosphorylation. Therefore, the link of erythropoiesis, regulation of iron homeostasis and metabolic reprogramming turns out to be crucial for the protection of the embryo from iron intoxication and its survival (Chakraborty et al., Cell Rep 2022). Currently, the comprehensive dynamic pathway models established for Epo-induced signal transduction in CFU-E cells and cellular model systems (Schilling et al., Mol Syst Biol 2009; Becker et al., Science 2010, Bachmann et al., Mol Syst Biol 2011, Adlung et al., Mol Syst Biol 2017) are adapted to the situation in patient derived erythroleukemia cells to unravel mechanisms that contribute to cancer progression and to propose promising intervention strategies.
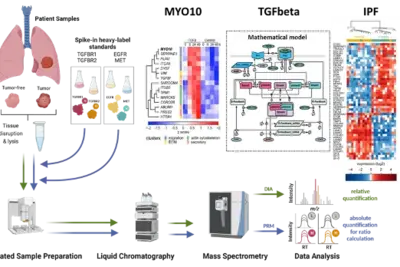
Deciphering tumor microenvironment interactions determining lung cancer development
Lung cancer remains a high incidence and high mortality disease. The most prevalent form of Non-Small Cell Carcinoma (NSCLC) is Lung Adenocarcinoma (LUAD) followed by Squamous Cell Carcinoma (LUSC). Transforming growth factor beta (TGFβ) has a dual role in cancer as it was uncovered as a tumor suppressor but also fosters epithelial-to-mesenchymal transition (EMT) in metastatic disease. Since high levels of TGFβ have been correlated with poor outcome in lung cancer and TGFβ has been identified as a key factor contributing to remodelling of the extracellular matrix (ECM), it is of particular importance to dissect its complex role in the tumor microenvironment in lung cancer. We showed by phenotypic studies and time-resolved next generation sequencing that TGFβ induces the upregulation of the non-muscle myosin MYO10. Interestingly, our studies revealed that the expression ratio of MYO10 in the tumor and the tumor-free tissue is prognostic for overall survival of LUSC patients and facilitates the prediction of chemotherapy response (Dvornikov et al., Sci Rep 2018). However, tumor progression not only depends on alterations in the cancer cell but is much affected by the communication within the tumor microenvironment. The importance of fibroblasts in lung cancer development is underscored by the observation that patients with idiopathic lung fibrosis (IPF) have an elevated risk to develop lung cancer. In alveolar type 2 cells of an IPF mouse model that harbors a conditional knockout of the ubiquitin ligase Nedd4-2 we observed an exacerbated TGFβ-induced SMAD signal transduction and an increased expression of components associated with remodeling of the extracellular matrix (Duerr et al., Nature Commun 2020). Currently, we are developing a dynamic pathway model of TGFβ signal transduction to quantitatively access the impact of ECM remodelling and of cell-cell communication on lung cancer cells. We explore patient derived samples to uncover mechanism-based biomarkers for early detection of lung cancer and sex-specific differences.
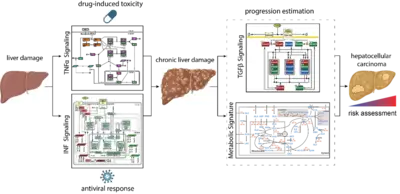
Mechanisms controlling the compensation of liver injury and towards model-based biomarkers for early detection of liver cancer
The liver has a central role in metabolism and detoxification. As the organ is constantly exposed to damaging insults, it has a remarkable capacity to regenerate and ensure survival. To maintain physiological function, these responses require tight regulatory mechanisms. In contrast to machine learning approaches that require large data sets, mechanistic models can be calibrated based on small data sets and can be employed as predictive tools seek to establish a causal relationship between inputs and outputs (D’Alessandro et al, Biochem J 2022). TGFβ is a key controlling factor coordinating the termination of liver regeneration. By model-based selection we identified the three most relevant SMAD-complexes in liver cells. This mathematical model suggests that elevated levels of SMAD proteins and SMAD2 phosphorylation are hallmarks of liver cancer (Lucarelli et al., Cell Syst 2018). Another factor implicated in the regulation of liver regeneration is tumor necrosis factor alpha (TNFα). We showed by dynamic pathway modelling that that multiple parameters in the TNFα network are affected by the non-steroidal anti-inflammatory compound Diclofenac leading to amplified toxicity and defining the toxicity threshold. (Oppelt et al. NPJ Syst Biol Appl 2018). Besides drugs, hepatotropic viruses such as hepatitis B virus (HBV) or hepatitis C virus (HCV) can cause liver damage and trigger interferon alpha (IFNα) antiviral responses. Dynamic pathway modeling enabled us to resolve the molecular mechanisms mediating hypersensitization or desensitization of the pathway. Adaptation of the mathematical model for individual patients in a virtual patient cohort revealed patient-specific predictors for pathway desensitization (Kok et al, Mol Syst Biol 2020). Due to the key role of the liver in metabolism a major interest is to elucidate interfaces between signal transduction involved in liver regeneration and metabolism. In a first step we contributed to a genome scale model that identified a high amino acid consumption rate in hepatoma cells and pointed to the importance of glutamate excretion, representing a potential cancer-specific intervention (Nilsson et al., Proc Natl Acad Sci U S A 2020). Currently, a major emphasis is on addressing the importance of remodelling of the ECM and the metabolic state on cancer progression and these events are reflected in the blood plasma of patients to facilitate early detection of liver cancer when surgical resection with curative intend is still possible.
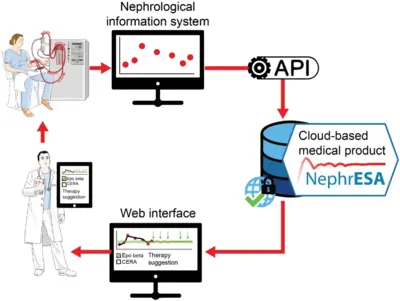
Application of dynamic pathway modelling for personalized medicine
The generation of high-quality quantitative data and the development of dynamic pathway models allows us to unravel disease promoting mechanisms and thus opens novel possibilities for early detection of cancer and personalized optimization of treatment. Our pioneering work on mathematical modeling of the interaction of the hormone Epo with its cell surface receptor EpoR on erythroid progenitor cells (Becker et al. Science 2010) serves as a paradigm. Anemia is characterized by low hemoglobin levels in the blood due to reduced numbers of red blood cells (erythrocytes) and occurs frequently in the context of chronic diseases such as latest stage of lung cancer or chronic kidney disease. The main cofounders are inflammation and chemotherapy in lung cancer and the lack of Epo production in the kidney, inflammation and uremic toxins in the case of chronic kidney disease. Just in Germany this affects per year more than 100,000 chronic kidney patients and up to 40,000 lung cancer patients. For these patients the anemia impairs quality of life, compromises palliative treatments and can lead to events with lethal outcome such as lung embolism, thrombosis or cardiac adverse events. The guidelines are generic for each disease although patients are very different. The lack of markers for the response of the patient to the different therapeutically employed Epo derivatives (Erythropoiesis Stimulating Agents; ESAs) often leads sub-optimal dosing or sporadically to overdosing. The resulting hemodynamic stress has been correlated with thrombovascular and cardiac adverse events. We developed a mechanism-based multiscale dynamic pathway model calibrated with pharmacokinetic and pharmacodynamics data of individual patients to assist clinical decisions in the personalization of anemia treatment in lung cancer and chronic kidney disease (patents). This mathematical model is capable to propose optimized personalized interventions for anemia management employing a minimal effective dose of ESAs and/or blood transfusions leading to safer hemodynamic profiles, and eventually a reduction in the risk of adverse events and mortality.
Team
-
Prof. Dr. Ursula Klingmüller
Division Leader
-
Yomn Abdullah
Doctoral Student
-
Annalisa Addante
Postdoctoral Researcher
-
Sandra Bonefas
-
Dr. Sebastian Burbano De Lara Carrillo
Postdoctoral Researcher
-
Katharina Büchner
-
Simone Clas
Doctoral Student
-
Kyra Fischer
-
Franziska Gödtel
-
Barbara Helm
-
Elisa Holstein
PhD Student
-
Martina Kegel
-
Daniel Kempter
Master's Student
-
Nicola Kunz
-
Dr. Nantia Leonidou
Postdoctoral Researcher
-
Caroline Lohoff
-
Till Möcklinghoff
-
Christina Mölders
PhD Student
-
Benedikt Niedermaier
-
Akane Ogawa
Visiting Bachelor's Student
-
Dr. Agustin Rodriguez Gonzalez
Postdoctoral Researcher
-
Tobias Roth
-
Michelle Sadlowski
-
Dr. Marcel Schilling
Deputy of Division
-
Lisa Strotmann
-
Katerina Sulková
PhD Student
-
Cong Quan Ta
PhD Student
-
Florian Tichawa
-
Leonie Wilhelm
-
Dr. Piotr Zadora
Postdoctoral Researcher
-
Waheb Hasan Zaidi
Selected Publications
Sebastian Burbano de Lara, Svenja Kemmer, Ina Biermayer, Svenja Feiler, Artyom Vlasov, Lorenza A D’Alessandro, Barbara Helm, Christina Mölders, Yannik Dieter, Ahmed Ghallab, Jan G Hengstler, Christiane Körner, Madlen Matz-Soja, Christina Götz, Georg Damm, Katrin Hoffmann, Daniel Seehofer, Thomas Berg, Marcel Schilling, Jens Timmer, and Ursula Klingmüller
Erythropoietin-driven dynamic proteome adaptations during erythropoiesis prevent iron overload in the developing embryo.
Sajib Chakraborty, Geoffroy Andrieux, Philipp Kastl , Lorenz Adlung, Sandro Altamura, Martin E. Boehm, Luisa E. Schwarzmüller, Yomn Abdullah, Marie-Christine Wagner, Barbara Helm, Hermann-Josef Gröne, Wolf D. Lehmann, Melanie Boerries, Hauke Busch, Martina U. Muckenthaler, Marcel Schilling, and Ursula Klingmüller.
Cell-to-cell variability in JAK2/STAT5 pathway components and cytoplasmic volumes defines survival threshold in erythroid progenitor cells.
Lorenz Adlung, Paul Stapor, Christian Tönsing, Leonard Schmiester, Luisa E Schwarzmüller, Lena Postawa, Dantong Wang, Jens Timmer, Ursula Klingmüller, Jan Hasenauer, Marcel Schilling.
Disentangling molecular mechanisms regulating sensitization of interferon alpha signal transduction.
Frédérique Kok, Marcus Rosenblatt, Melissa Teusel, Tamar Nizharadze, Vladimir Gonçalves Magalhães, Christopher Dächert, Tim Maiwald, Artyom Vlasov, Marvin Wäsch, Silvana Tyufekchieva, Katrin Hoffmann, Georg Damm, Daniel Seehofer, Tobias Boettler, Marco Binder, Jens Timmer, Marcel Schilling, and Ursula Klingmüller.
All Publications
Get in touch with us
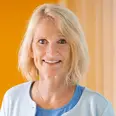
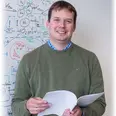