Radiology
- Imaging and Radiooncology

Prof. Dr. Heinz-Peter Schlemmer
Leitung Abteilung Radiologie
Goal of the division of Radiology is to develop, validate and clinically implement imaging methods for improved cancer diagnostics and therapy and to transfer the technologies into the society and healthcare system. The division is rooted within the comprehensive research topic „Imaging and Radiooncology“ with its strengths in medical physics, radiopharmacy, (radio)biology and bioinformatics, and complements the shared infrastructure with expertise to conduct clinical trials.
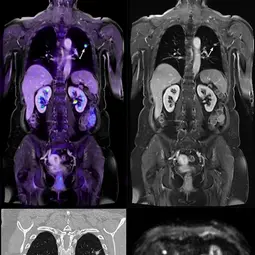
Our commitment to patients and research
The well-being of our patients is our top priority. Our team guarantees empathetic, professional care and uses state-of-the-art diagnostic procedures.
Innovative research & interdisciplinary collaboration
Thanks to close cooperation with specialist and research departments in our "Imaging and Radiation Oncology" focus area, we pool scientific expertise and develop new imaging procedures.
In collaboration with our partners, we are continuously improving the methodology of:
- Sonography (ultrasound)
- Computed tomography (CT)
- Magnetic resonance imaging (MRI)
- Positron emission tomography (PET)
These procedures are tested and optimized in clinical studies in order to provide patients with even more precise diagnostics and therapy.
The latest generation of technology
State-of-the-art systems are available for our research and diagnostics, including
- Ultra-high-field MRI (7 Tesla)
- Dual-energy CT
- PET-MRI of the latest generation
- PET-CT of the latest generation in cooperation with the KKE Nuclear Medicine and the Junior Research Group Translational Molecular Imaging in Oncological Therapy Monitoring
Cooperation with leading institutions
Our clinical-scientific studies are carried out in cooperation with
- Heidelberg University Hospital
- National Center for Tumor Diseases (NCT)
- Medical technology & pharmaceutical industry partners
- Other renowned research institutions & clinics
Our mission: Innovative imaging for more precise diagnostics and better patient care.
Selected publications
Wennmann M, Ming W, Bauer F, Chmelik J, Klein A, Uhlenbrock C, Grözinger M, Kahl KC, Nonnenmacher T, Debic M, Hielscher T, Thierjung H, Rotkopf LT, Stanczyk N, Sauer S, Jauch A, Götz M, Kurz FT, Schlamp K, Horger M, Afat S, Besemer B, Hoffmann M, Hoffend J, Kraemer D, Graeven U, Ringelstein A, Bonekamp D, Kleesiek J, Floca RO, Hillengass J, Mai EK, Weinhold N, Weber TF, Goldschmidt H, Schlemmer HP, Maier-Hein K, Delorme S, Neher P
von Knebel Doeberitz N, Kroh F, Breitling J, König L, Maksimovic S, Graß S, Adeberg S, Scherer M, Unterberg A, Bendszus M, Wick W, Bachert P, Debus J, Ladd ME, Schlemmer HP, Korzowski A, Goerke S, Paech D
Tavakoli AA, Hielscher T, Badura P, Görtz M, Kuder TA, Gnirs R, Schwab C, Hohenfellner M, Schlemmer HP, Bonekamp D
Sedlaczek OL, Kleesiek J, Gallagher FA, Murray J, Prinz S, Perez-Lopez R, Sala E, Caramella C, Diffetock S, Lassau N, Priest AN, Suzuki C, Vargas R, Giandini T, Vaiani M, Messina A, Blomqvist LK, Beets-Tan RGH, Oberrauch P, Schlemmer HP, Bach M, CCE – Imaging Task Force
Get in touch with us
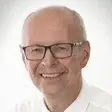