Medical Image Computing Research
- Helmholtz Metadata Collaboration (HMC) - Hub Health
- Predicting Immunotherapy Outcome of Lung Cancer Patients by Composite Radiomics Signatures in CT Scans
- Leveraging Similarity between Learned Representations
- Deep Learning for Discovering Predictive Biomarkers in Glioblastoma Imaging
- Learning from multicentric medical imaging data
- Self-configuring Medical Object Detection and Instance Segmentation
- Anomaly Detection Using Unsupervised Learning for Medical Images
- Multitask Segmentation using partially annotated datasets
- Transformers and self-attention in medical image anaylsis
- Characterization and prediction of COPD as a comorbidity from computed tomography imaging
- Large scale image analysis and computational pathology
- Helmholtz Federated IT Services (HIFIS) Consulting
- Addressing misalignment for enhanced prostate MRI analysis
- Joint Imaging Platform
- Self-Supervised Representation Learning in Medical Image Analysis
- Medical Imaging Interaction Toolkit (MITK)
- MITK Modelfit and Perfusion
- Research data management and automated processing
- Automatic Image Analysis in Patients with Multiple Myeloma
- End-To-End Text Classification of Multi-Insitutional Radiology Findings
- Intraoperative assistance for mobile C-arm positioning
- Automatic image-based pedicle screw planning
- Radiological Cooperative Network - RACOON
- Computational analysis of subclinical comorbidities in clinical routine CT data
- Temporal and Global Consistency Enforcing Segmentation for Real World Radiological Applications
- Assisting Breast Cancer Decisions using a Software System utilizing Deep Learning Trained on Diffusion Weighted MRI Data
- Trustworthy Federated Data Analytics (TFDA)
- VISSART: VISualiSation And Ranking Toolkit (joint project with IMSY division)
- Hierarchical instance segmentation of mineral particles for automated particle composition identification
- DCE/DSC Lexicon as part of the „Open Science Initiative for Perfusion Imaging“
- Digital Cancer Prevention
- Kaapana
- HiGHmed
- CCE-DART
- CSI-HD
- Hyppopy
Helmholtz Metadata Collaboration (HMC) - Hub Health
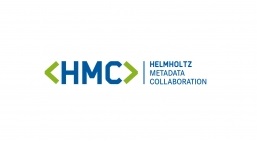
© dkfz.de
The Helmholtz Metadata Collaboration Platform develops concepts and technologies for efficient and interdisciplinary metadata management spanning the Helmholtz research areas Energy, Earth and Environment, Health, Matter, Information, Aeronautics, Space and Transport. As HMC Hub Health, we support researchers and clinicians in structuring, standardizing, and expanding the collection of metadata to facilitate the re-use, interoperability, reproducibility, and transparency of their data.
More information: https://www.helmholtz-metadaten.de
Predicting Immunotherapy Outcome of Lung Cancer Patients by Composite Radiomics Signatures in CT Scans
Leveraging Similarity between Learned Representations
Deep Learning for Discovering Predictive Biomarkers in Glioblastoma Imaging
Learning from multicentric medical imaging data
Self-configuring Medical Object Detection and Instance Segmentation
Anomaly Detection Using Unsupervised Learning for Medical Images
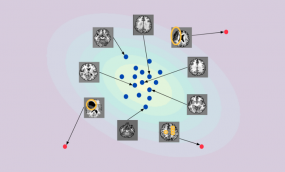
© dkfz.de
An assumption-free automatic check of medical images for potentially overseen anomalies would be a valuable assistance for a radiologist. Deep learning and especially generative models such as Variational Auto-Encoders (VAEs) have shown great potential in the unsupervised learning of data distributions. By decoupling abnormality detection from reference annotations, these approaches are completely independent of human input and can therefore be applied to any medical condition or image modality. In principle, this allows for an abnormality check and even the localization of parts in the image that are most suspicious.
Multitask Segmentation using partially annotated datasets
Transformers and self-attention in medical image anaylsis
Transformer based architectures have been introduced to computer vision with the Vision Transformer (ViT). Since then, research efforts have sought to replicate the breakthroughs in natural language processing in vision based tasks such as classification, detection and segmentation using transformers. Medical image segmentation has seen its share, with some research incorporating ViT backbones while others using hierarchical feature search alongside transformer layers promising high performance. This project seeks to explore the effectiveness of transformer based architectures, in comparison to well-understood convolutional nets, in the face of realistic dataset sizes in medical image analysis.
Characterization and prediction of COPD as a comorbidity from computed tomography imaging
Chronic Obstructive Pulmonary Disease (COPD) is a common lung disease characterized by persistent or recurrent respiratory symptoms. Different patterns ("phenotypes") of lung damage are observed with different consequences for individual therapy, e.g. the destruction of the alveolar sac (emphysema) or bronchial wall thickening and obstruction with mucus (airway disease). Medical air-flow lung function tests typically fail to detect subtle changes within the lungs or even sub-regions of the lung. Beyond visual inspection of computed tomography (CT), quantitative CT analysis using computer-aided detection and deep learning techniques promises more insight into the lung and its reactions to the disease or medication. We aim to further analyze the CT images by exploring unseen patterns and clusters by deep learning in order to find new methods for the classification and monitoring of COPD.
Large scale image analysis and computational pathology
Helmholtz Federated IT Services (HIFIS) Consulting
HIFIS offers free-of-charge consulting as a service to research groups under the Helmholtz umbrella. We help you to deal with specific licensing issues, pointing out solutions on how to improve your software or setting up a new projects. We are also very happy to discuss other software engineering topics such as software engineering process in general. We are a small team that tries to help as many researchers and research groups as possible across all Helmholtz institutes.
Addressing misalignment for enhanced prostate MRI analysis
Joint Imaging Platform
Within the German Cancer Consortium (DKTK) the Joint Funding Project “Joint Imaging Platform” will establish a distributed IT infrastructure for image analysis and machine learning in the member institutions. It will facilitate pooling of analysis methods that can be applied in an automated and standardized manner to patient data in the different centers, allowing for unprecedented cohort sizes. The biggest research challenge is the combination, aggregation and distribution of training data, processes and models for non-shareable sensitive data as well as the validation of quantitative imaging biomarkers across a multi-institutional consortium. On the implementation side we investigate distributed learning methods as well as latest private cloud technologies for a robust deployment of data management and processing. More information
Self-Supervised Representation Learning in Medical Image Analysis
Medical Imaging Interaction Toolkit (MITK)
Cancer Research Center since 2002 with contributions and users from an
international community in research and industry.
MITK Modelfit and Perfusion
Model fitting plays a central role in the quantitative analysis of medical images. One prominent example is dynamic contrast-enhanced (DCE) MRI, where perfusion-related parameters are estimated using pharmacokinetic modelling. Other applications include mapping the apparent diffusion coefficient (ADC) in diffusion weighted MRI, and the analysis of Z-spectra in chemical exchange saturation transfer (CEST) imaging.
The ready-to-use model fitting toolbox is embedded into MITK and provides tools for model-fitting (ROI-based or voxel-by-voxel), fit evaluation and visualization. Any fitting task can be performed given a user-defined model. Being part of MITK, MITK-ModelFit applications can be easily and flexibly incorporated into pre-and post-processing workflows and offer a large set of interoperability options and supported data formats.
A special emphasis is put on pharmacokinetic analysis of DCE MRI data. Here, a variety of pharmacokinetic models is available. In addition tools are offered for arterial input function estimation and conversion from signal to concentration.
Research data management and automated processing
Additionally to the interactive processing focused in MITK, today's research questions often require the standardized and automated processing and easy data access while reducing emerging hassles of data transfer, data protection and data storage. We provide scientific cloud and platform solutions and evaluate new exploration and processing capabilities to medical imaging researchers.
Automatic Image Analysis in Patients with Multiple Myeloma
End-To-End Text Classification of Multi-Insitutional Radiology Findings
Intraoperative assistance for mobile C-arm positioning
Intraoperative imaging guides the surgeon through interventions and leads to higher precision and reduced surgical revisions. For evaluation purposes the surgeon needs to acquire anatomy-specific standardized projections. We aim to replace the current manual positioning procedure of the C-arm involving continuous fluoroscopy by an automatic procedure, thereby reducing the dose and time requirement. We tackle this problem employing data simulation techniques and deep learning based methods.
Automatic image-based pedicle screw planning
Radiological Cooperative Network - RACOON
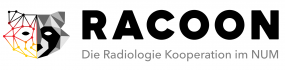
© dkfz.de
The department of medical image computing provides its expertise in building federated machine learning infrastructures. It contributes the Kaapana software platform allowing federated learning and image analysis as well as method sharing between the partners by providing support to executed containerized methods either on-site as part of the local RACOON-Nodes or in the central component as part of RACOON-Central.
Computational analysis of subclinical comorbidities in clinical routine CT data
Extensive research has been conducted in the field of image-based computational analysis of major clinical diseases. By contrast, little is known about the potential variety of interrelations between typical co-occuring pathologies. During clinical routine, diagnosis and treatment are normally targeted at a primary disease, while co-occuring pathologies often remain undetected and underdiagnosed, despite their expected substantial effect on overall prognoses and treatment outcomes. This project focuses on a more holistic analysis of imaging data from clinical routine, as a means to automatically detect and quantify an expected variety of co-occuring diseases. Therefor, a well-defined subset of pathologies and datasets at University Hospital Heidelberg serves as a foundation for the development of learning based image analysis algorithms, with the goal to promote a deeper understanding of comorbidities encountered in clinical practice. For seamless translation of research methods into the clinical workflow and for future scalability of the project, an integrated system for automated algorithm deployment is developed based on the joint imaging platform (JIP).
Temporal and Global Consistency Enforcing Segmentation for Real World Radiological Applications
State-of-the-art segmentation frameworks show impressive performance with dice scores comparable to human inter- and intra-rater variability. However, these models frequently fail with severe and unexpected errors when being brought into the clinical environment. In this project a model will be developed which will incorporate the insights obtained from the analysis of the performance of current methods, as well as temporal and global information of samples in order to improve and stabilize the generated segmentations.
Assisting Breast Cancer Decisions using a Software System utilizing Deep Learning Trained on Diffusion Weighted MRI Data
Breast cancer is the most invasive cancer for women throughout the world, in both developed and developing countries. This project aims to create robust models for breast lesion detection and classification using diffusion-weighted MR images and to produce a software platform, up to the high standards of market medical products, that can interactively assist clinical decision making by providing valuable feedback and diagnostic suggestions. The aspiration is that the deep learning model will be able correctly identify and classify malignant lesions with high sensitivity, while minimizing false positive results which are common in standard clinical screening practice.
Trustworthy Federated Data Analytics (TFDA)
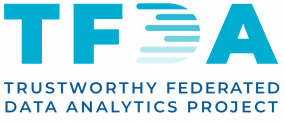
© dkfz.de
Artificial intelligence in medical research can accelerate the acquisition of scientific knowledge, facilitate early diagnosis of diseases and support precision medicine. The necessary analyzes of large amounts of health data can only be achieved through the cooperation of a large number of institutions. In such collaborative research scenarios, classic centralized analysis approaches often reach their limits or fail due to complex security or trust requirements.The goal of the multidisciplinary team in the Trustworthy Federated Data Analytics (TFDA) project is therefore not to store the data centrally, but instead to bring the algorithms for machine learning and analysis to the data, which remains local and decentralized in the respective research centers. As a proof of concept, TFDA will establish a pilot system for federated radiation therapy studies and deal with the necessary technical, methodological and legal aspects to ensure the quality and trustworthiness of the data analysis and to guarantee the privacy of the patients.
More information: https://tfda.hmsp.center/
VISSART: VISualiSation And Ranking Toolkit (joint project with IMSY division)
VISSART is an open-source framework (based on challengeR) for analyzing and visualizing challenge/benchmarking/algorithm results. It offers a set of tools for comprehensive analysis and visualization of method results. It applies a number of simulated and real-life computations such as visualizing assessment data, ranking robustness, and ranking stability, to demonstrate specific strengths and weaknesses of various algorithms. Furthermore, it also supports single-task and multi-task challenges. Thanks to this online version, there is no need of installing interpreters, necessary packages, libraries, etc. We facilitate the use of the challengeR framework to developers not familiar with the R language.
Hierarchical instance segmentation of mineral particles for automated particle composition identification
The analyzation and identification of particle compositions plays a central role in increasing the effectiveness of ore processing and recycling techniques. These types of analyses are typically performed by crushing ores of unknown composition into particles, which are then placed in a synthetic resin embedding and CT-scanned in micron resolution. The resulting CT-scan is then manually analyzed in a time intensive and error-prone manner to identify the individual composition of multiple hundreds of particles.
The objective of this project is to automatically generate hierarchical instance segmentations of every particle in order to reduce the current time intensive approach and increase the correctness of the analysis.
DCE/DSC Lexicon as part of the „Open Science Initiative for Perfusion Imaging“
Perfusion-related quantities derived from dynamic contrast-enhanced (DCE) and dynamic susceptibility contrast (DSC) magnetic resonance imaging (MRI) are useful biomarkers of vascular function. To generate perfusion-related quantities, the acquired data is typically analyzed using a sequence of processes which define an image analysis pipeline. Currently, there is a lack of clear reporting guidelines and standardized nomenclature for applied perfusion analyses pipelines. This means that analysis steps are often not accurately captured, leading to user-variability in reporting, which fundamentally limits reproducibility of perfusion-based research.The Open Source Initiative for Perfusion Imaging (OSIPI) is an initiative of the International Society for Magnetic Resonance in Medicine (ISMRM) perfusion study group with the mission is to promote the sharing of perfusion imaging software, improve the reproducibility of perfusion imaging research. As part of OSIPI a consensus-based DCE/DSC lexicon and a reporting framework are developed with the aim to improve reproducibility and in perfusion image analysis.
Digital Cancer Prevention
To develop a research-supporting risk prediction platform for the National Cancer Prevention Center, we are currently assembling an interdisciplinary digital cancer prevention team. The focus of the working group is on the development of a specific and evidence-based portal for the individual calculation of personal cancer risk. In doing so, existing prediction models will be validated, curated and merged according to a standardized procedure. Interested citizens should be able to use the portal to assess their individual cancer risk and receive information adapted to their personal cancer risk. For example, demographic data, information on lifestyle, family history, and results of previous tests can be included in the calculation. At the same time, these data are used to further optimize the prediction models and maintain a continuously high level of performance. In the long term, the aim is to develop a research-capable platform for sustainable data collection and access to research data in modern prevention research.
Kaapana
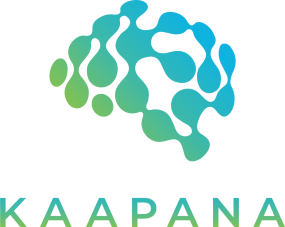
© dkfz.de
Kaapana is a technology platform for Distributed Computational Image-based PHEnotyping and Radiomics. It is designed to establish a better link between clinical (imaging) data, computational power and methodical tools.
Kaapana supports single-institutional use, where it improves direct workflow integration of computing tools or analysis of meta data. However, Kaapana also scales to multi-institutional settings, where it scales with the computational resources, the sizes of cohorts and with the number of methods available. The federated computing capabilities are readily built in - so no centralization (neither for data nor for methods) is needed. Leveraging state of the art open source technologies we aim at a high interoperability with existing standards and solutions.
More information:
https://www.kaapana.ai/
https://github.com/kaapana/kaapana.
HiGHmed
HiGHmed is a highly innovative consortial project in the context of the "Medical Informatics Initiative Germany" that develops novel, interoperable solutions in medical informatics with the aim to make medical patient data accessible for clinical research in order to improve both, clinical research and patient care. Our image analysis technology (d:cipher) is part of the Omics Data Integration Center (OmicsDIC) that offers sophisticated technologies to process data and to access information contained in data - from genomics to radiomics. In HiGHmed we also improve the interoperability of image based information by working on the mapping between different important standards like DICOM, HL7 FHIR or OpenEHR.
More information: HiGHmed
CCE-DART
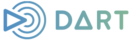
© dkfz.de
The EU-funded project CCE-DART (CCE Building Data Rich Clinical Trials) aims to develop novel methods for the design and implementation of newer, more efficient and effective clinical trials. At DKFZ experts from five departments contribute to this goal. This includes the department of medical image computing which provides its expertise in federated image analysis to build a data sharing and analysis platform. The platform will be based on the Kaapana technology platform and will allow researchers to find relevant imaging data and perform federated image analysis.
More information: https://cce-dart.com/
CSI-HD
To make forensic radiology feasible, we develop workflows and processes that combine local and remote radiological infrastructure as well as latest technologies of medical image processing. To minimize required resources at image acquisition sites, we design automated image processing pipelines and secure data transfers to minimally occupy human resources and minimally interfere with previously existing on-site routine workflows.
This project is a cooperation between DKFZ, Institute for Legal and Traffic Medicine (University Clinic HD) and Institute for Anatomy (University HD).
Hyppopy
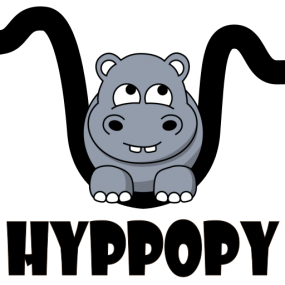
© dkfz.de
Hyppopy is a python toolbox for blackbox-function optimization providing an easy to use interface for a variety of solver frameworks. Hyppopy gives access to gridsearch, random and quasirandom, particle and bayes solvers. The aim of Hyppopy is making hyperparameter optimization as simple as possible (in our cases e.g. for the optimization of image processing pipelines or machine learning tasks). It can be easily integrated in existing code bases and provides real-time visualization of the parameter space and the optimization process via a visdom server. The internal design is focused on extensibility, ensuring that custom solver and future approaches can be integrated.