Surgical Simulation on Cyber-physical Organ Models
Participating Researchers
Current members: Ann-Sophia Müller, Do Yeon Kim, Moonkwang Jeong, Lalith Boggaram-Naveen
Former members: Dr. med. Xiangzhou Tan, Dr. Eunjin Choi, Nurcemal Atmaca, Dandan Li
Artificial Intelligence (AI) has revolutionized many fields, such as computer vision and natural language processing. These beneficial areas share the common features that the available data are abundant, standardized, accessible and relatively cheap to acquire. However, when similar machine learning approaches are applied to physical or hardware problems, one of the major challenges is how to obtain a large amount of data under realistic experimental conditions. Contrary to photos and texts that are digitalized and standardized, the data from medical field are often sparse, non-standardized, inaccessible, time-intensive and expensive to acquire. The sensing and modeling of data on a realistic model system is the key to many design problems. The main objective of this project is to develop the cyber-physical twin of human organs that combines the advantages of physical and cyber models, to facilitate the sensing of hard-to-acquire biomedical data under realistic conditions and to allow the analysis and modeling using this data.
A hybrid surgical simulator for endoscopic training
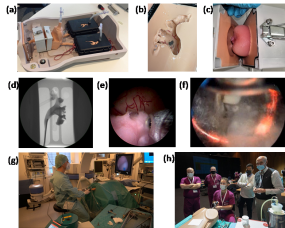
Figure 1. The Endo Urology Trainer. (a-c) The integrated hardware system: (a) System overview; (b) The kidney model; (c) Resectable soft prostate model. (d-f) Example minimally-invasive procedures: (d) X-ray imaging; (e) Enucleation and morcellation; (f) Electrocautery. (g-h) Testing of the models by medics: (g) In an operation room; (h) A medical workshop (a-g, unpublished results; h, the photo is provided by R. Wolf GmbH).
© dkfz.de
Key Collaborator: Prof. Arkadiusz Miernik, Department of Urology, University Hospital Freiburg
We developed a hybrid surgical simulator – a combination of realistic physical organ phantoms and AI-based virtual environment for endourological training. The physical part represents a full urinary tract model (Fig. 1a-c), which integrates two kidneys, a bladder and a prostate phantom. The system "Endo Urology Trainer" (Quantitative Surgical GmbH, Mannheim, Germany) not only represents the human anatomy but also mimics the mechanical properties of soft tissues and detailed blood vessels on the internal lumen walls (Fig. 1d-f). Endourological procedures were performed with clinical endoscopes (Fig. 1g) to validate the model. We also developed an AI algorithm to automatically detect lesions and to quantitatively evaluate the surgical outcome for feedbacks [ref 1-3].
Another example is that we created the world's first multi-layer prostate model based-on customized hydrogel materials that can be resected with real instruments [ref 4]. The trainer has been applied in the Canadian Association of Urology for training of a new laser therapy (Fig. 1h). Besides, our trainers have been widely used in international urological workshops. We estimate that ~100 urological surgeons have used our system and trained their endoscopic skills.
3D ultrasound scanning and image analysis system for surgical outcome evaluation
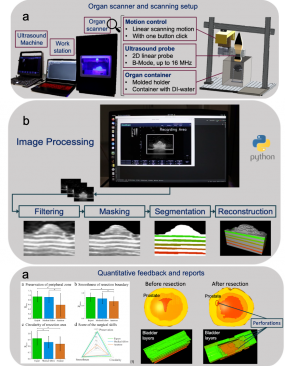
Figure 2. The organ scanner automated pipeline. (a) The customized scanning system, (b) Image stack acquisition and processing depicted for bladder tumor endoscopic surgery, (c) Evaluation and interactive feedback by 3D reconstructions. Evaluated parameters include smoothness, accuracy, and circularity of the resection. [ref 5]
© dkfz.de
Surgical training is more effective if the results can be evaluated in a quick and quantitative manner. We therefore developed a fully-automated pipeline for the quantitative outcome assessment in surgical trainings. Here, with a focus on the transurethral resection of the prostate (TURP) and the transurethral removal of bladder tumor (TURBT). The procedures can be performed on the aforementioned realistic phantom within the "Endo Urology Trainer". A customized ultrasound scanner is developed to record the resected part of the organ phantoms. The phantoms are engineered with high imaging contrast, which enables a subsequent evaluation process based on image segmentation, reconstruction, parameter extraction and evaluation (Fig. 2). The 3D-visualization and the performance metrices allow surgeons to pin-point mistakes, provide quantitative feedback and accelerate surgical training [ref 5].
Key publications related to the topic
1. Kim et al. (2022): A high-fidelity artificial urological system for the quantitative assessment of endoscopic skills, J. Funct. Biomater., 13(4)301, DOI: 10.3390/jfb13040301
2. Kim et al. (2021): Computer-assisted quantitative assessment of endo. skills based on a uro-logical phantom, 55th DGBMT Ann. Conf. on Biomed. Eng., Talk, 66, S212-217, Link to abstract.
3. Kim et al. (2022): A hybrid surgical simulator for interactive endoscopic training, IEEE Inter. Conf. of the Engineering in Medicine and Biology Society (EMBC), Poster, DOI: 10.1109/EMBC48229.2022.9871697
4. Choi et al. (2020): A High-Fidelity Phantom for the Simulation and Quantitative Evaluation of Transurethral Resection of the Prostate, Ann. Biomed. Eng., 48, 437-446. https://link.springer.com/article/10.1007/s10439-019-02361-7
5. Müller A.-S., et al. (2023): Surgical training of tumor resection on hydrogel models with quan-titative assessment based on 3D ultrasound scanning. DKFK German Cancer Research Con-gress (GCRC). Poster