Functional
Genome Analysis (B070)
Deutsches
Krebsforschungszentrum,
Im Neuenheimer Feld 580
D-69120
Heidelberg,
Germany. |
 |
..
.
..
Affinity
Proteomics
- Antibody
Microarrays
..
..
...
...
As a
consequence of
large-scale genomic sequencing, strong interest has
emerged in
analysing the function of the DNA-encoded information on a similarly
global
scale. However, many aspects of modulation and regulation of cellular
activity
cannot be investigated at the level of nucleic acids but require an
analysis of
the proteome.
..
The
complexity
in the human proteome is expected to range from one hundred thousand to
several
million different protein molecules, all based on the about 22,000
protein-encoding genes. The
situation is additionally complicated by the facts that not for every
protein of multicellular organisms the function is
known and
that a protein may have different functions dependent on structure
variations,
interacting partners, location and time of expression. Also, the
dynamic range of protein expression is very large indeed.
..
Various
mass-spectrometry-based processes exist for
a powerful analysis of
proteins of an organism or tissue. Also, assays such as
yeast-two-hybrid analyses in all their
facets permit global studies for
the
identification of interaction partners. As a third approach, affinity
proteomics
has an enormous potential in a
global characterisation of molecule mixtures at
the
protein level.
Knowledge of
genomic sequences and transcriptional profiles do not suffice for a
reliable
description of actual protein expression, let alone an analysis of
protein structures and
biochemical activities or a
quantitative examination of
protein-protein interactions. This kind of information, however, is
crucial for an
understanding of the molecular biology of
cells, tissues or whole
organisms
and has a broad biotechnical and medical potential. We perfom
such analyses on a relatively large-scale with
nevertheless high reproducibility, a near-single-molecule sensitivity,
and an accuracy that is superior to ELISA-based
assays.
Antibody
microarrays:
Utilising
antibody
microarrays that currently consist of some 3,400 antibodies, we pursue
the analysis of studying variations in actual
protein abundance, isoform occurrence and other structural variations. Basic
technical
processes are understood in much
detail, such as
the choice of appropriate surfaces, the effect of kinetics
and mass transport and
labelling procedures as well as many other aspects. Detailed
protocols are available that allow reproducible and reliable
analysis of expression variations on complex protein extracts from
tissues, cells or body liquids down to
attomolar concentrations. Antibody
generation and selection was and is performed in collaborations
with companies as well as academic partners, partly within consortia
that aim at the creation of well-characterised and specific antibodies
or other binders (e.g., Affinomics). In addition,
improved preparation of
protein extracts proved
crucial for success. The current
set-up was and is used in various projects, frequently combining the
information on protein levels
with other
data. Also, quantification of the results is performed.
Protein
microarrays:
We
utilise protein
microarrays
containing mostly full-length molecules for the
investigation of protein-interactions
in a quantitative manner. Microarray production is
done by in situ synthesis by an in vitro
transcription and translation process on the microarrays, starting from
full-length cDNAs or gene-specific PCR-products. Protein
interaction of all kinds as
well as the influence of co-factors such as small molecules are
studied this
way. The most complex protein array produced so far contained some
14,000
individual proteins. The set-up
is used in various projects and on the proteome of several organisms.
Personalised
proteomics:
In
a recently completed technical development, we added to the in situ protein production a
process that allows to present on the microarray the proteins in
exactly the conformation as they occur in tissues or other samples of
individual patients, reflecting all mutations or splice variants that
are specific for the particular sample. Thereby, particularly the
effects of individual variations on protein interaction - with other
proteins, nucleic acids or small compounds - can be studied in a
quantitative manner.
|
|
|
|
|
Zhang
et
al.
(2023) Clin. Cancer
Res., in press. |
 |
|
Syafrizayanti et
al. (2014) Exp. Rev. Prot. 11,
107. |
 |
|
|
|
|
Brindl et
al. (2022) Cancers 14, 3562. |
 |
|
Marzoq et
al. (2013) J. Biol.
Chem. 288,
32517. |
 |
|
|
|
|
Roth et
al. (2021) Annals
Surgery 273, e273-e275. |
 |
|
Lueong et
al. (2013) J. Prot.
Bioinf. 07, 004. |
 |
|
|
|
|
Morath et
al. (2020) J. Clin.
Invest. 26, 2364-2376. |
 |
|
Schröder et
al. (2013) Proteomics
Clin. Appl. 7, 802. |
 |
|
|
|
|
Ghassem-Zadeh et
al. (2020) Int. J. Mol.
Sci. 130, 2403. |
 |
|
Hoheisel et
al. (2013) Proteomics
Clin. Appl. 7, 8. |
 |
|
|
|
|
Jeske et
al. (2020) Cancer
Epidem. Biomarkers Pref. 29,
2235-2242. |
 |
|
Alhamdani et
al. (2012) J. Proteomics 75, 3747. |
 |
|
|
|
|
Weidmann et
al. (2019) CELL Chem.
Biol. 26, 645-651. |
 |
|
Friedrich et
al. (2011) Proteomics 11, 3757. |
 |
|
|
|
|
Marzoq et
al. (2019) Sci. Rep. 9,
5303. |
 |
|
Schmidt et
al. (2011) J. Prot. Res. 10, 1316. |
 |
|
|
|
|
Hufnagel et
al. (2019) Bio-protocol 9, e3152. |
 |
|
Schröder et
al. (2011)
Protein
Micoarrays - Meth. Mol. Biol.,
203. |
 |
|
|
|
|
Goerke et
al. (2018) NMR Biomed. 31, e3920. |
 |
|
Alhamdani & Hoheisel (2011) Mol. Anal.
& Genome Disc., Wiley, 219. |
|
|
|
|
|
Kunz et
al. (2018) Sci. Rep. 8,
7934. |
 |
|
Sill et al. (2010) BMC
Bioinformatics 11, 556. |
 |
|
|
|
|
Hufnagel et
al. (2018) Sci. Rep. 8, 7503. |
 |
|
Alhamdani et al. (2010) Proteomics 10, 3203. |
 |
|
|
|
|
Kunz et
al. (2017) BBA-Gen.
Subjects 1861, 2196-2205. |
 |
|
Schröder et
al. (2010) Antibody
Engineer., Vol. 2,
Springer, 429. |
 |
|
|
|
|
Mustafa et
al. (2017) Oncotarget
8,
11963-11976. |
 |
|
Alhamdani et al. (2010) J. Prot. Res. 9, 963. |
 |
|
|
|
|
Syafrizayanti et
al. (2017) Sci. Rep. 7,
39756. |
 |
|
Schröder et
al. (2010) Mol. Cell.
Prot. 9,
1271. |
 |
|
|
|
|
Kamhieh-Milz et
al. (2016) J. Proteomics 150, 74-85. |
 |
|
Gloriam et
al. (2010) Mol. Cell.
Prot. 9,
1. |
 |
|
|
|
|
Bakdash et
al. (2016) Cancer Res. 76, 4332-4346. |
 |
|
Alhamdani et al. (2009) Genome Med. 1,
68. |
 |
|
|
|
|
Loeffler et
al. (2016) Nature Comm. 7, 11844. |
 |
|
Börner et
al. (2009) BioTechniques 46,
297. |
 |
|
|
|
|
Sill et
al. (2016) Microarrays 5, 19. |
 |
|
Taussig et al. (2007) Nature
Meth. 4, 13. |
 |
|
|
|
|
Kibat et
al. (2016) New
Biotechnol. 33, 574-581. |
 |
|
Kusnezow et
al. (2007) Proteomics 7,
1786. |
 |
|
|
|
|
Bal et
al. (2016) Br. J.
Haematology 4, 602-615. |
 |
|
Kusnezow et
al. (2006) Mol. Cell.
Prot. 5, 1681. |
 |
|
|
|
|
Nijaguna et
al. (2015) J. Proteomics 128, 251-261. |
 |
|
Angenendt et al.
(2006) Mol.
Cell. Prot. 5,
1658. |
 |
|
|
|
|
Mock et
al. (2015) Oncotarget 6, 13579-13590. |
 |
|
Kusnezow et
al. (2006) Proteomics 6,
794. |
 |
|
|
|
|
Betzen et
al. (2015) Proteomics Clin. Appl. 9, 342.
|
 |
|
Kersten et
al. (2005) Expert
Rev. Proteomics 2, 499. |
 |
|
|
|
|
Bradbury et
al. (2015) Nature 518,
27. |
 |
|
Kusnezow &
Hoheisel .(2003) J. Mol. Recognit. 16,
165. |
 |
|
|
|
|
Hoheisel (2014) labor&more 10/14, 10. |
 |
|
Kusnezow et
al. (2003) Proteomics 3,
254. |
 |
|
|
|
|
Srinivasan et
al. (2014) Proteomics 14, 1333. |
 |
|
Kusnezow &
Hoheisel (2002) BioTechniques 33, 14. |
 |
|
|
|
|
|
|
.
Blood-based
diagnosis and risk stratification of patients with intraductal
papillary mucinous neoplasm (IPMN) to decide on surgical intervention
Intraductal
papillary mucinous neoplasm (IPMN) is a
precursor of PDAC. Patients with low-grade dysplasia have a relatively
good
prognosis and are kept under surveillance to monitor disease
development,
whereas high-grade dysplasia and IPMN invasive carcinoma require tumour
resection. Diagnostic distinction of the two groups is difficult,
however. We
aimed to identify variations in protein concentration in peripheral
blood for
accurate discrimination. Sera from IPMN patients and healthy donors were
analysed on microarrays made of 2,977
antibodies. For microRNA biomarkers, a PCR-based screen was performed
and
biomarker candidates confirmed by quantitative PCR.
A support vector
machine
(SVM) algorithm defined classifiers, which were validated on a separate
sample
set. A panel of five proteins and three miRNAs could distinguish high-
and
low-risk IPMN with an accuracy of 97%. This is substantially better
than the
accuracy obtained in the same patient cohort by using the guideline
criteria
for decision-making on performing surgery or not. The precise
blood-based
diagnosis and risk stratification will improve patient management and
thus the
prognosis of IPMN patients. In addition to the main finding, highly
accurate
discrimination was also achieved between other patient subgroups.
Zhang
et
al.
(2023) Clin. Cancer
Res., in press. 
|
|
Figure legend: (Left) Diagnostic
performance of
clinical parameters to discriminate high-risk from low-risk IPMN
according to
current guidelines. (Right) Much
better results were obtained by a combined panel of 8 protein and miRNA
biomarkers. The results are presented as ROC curves and corresponding
AUC
values as determined in the training and validation cohorts,
respectively. |
.
|
..
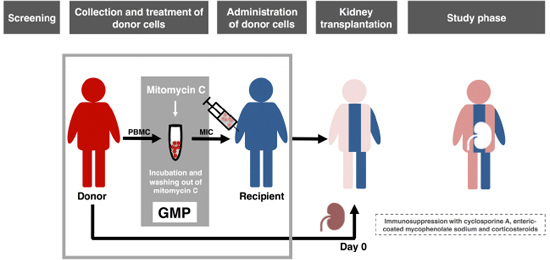
|
|
Phase-I trial
of donor-derived immune cell infusion in kidney transplantation
Preclinical
experiments have shown that donor blood cells, modified in vitro by
an
alkylating agent (MIC, modified immune cells), induced long-term
specific
immunosuppression against the allogeneic donor. In this phase-I trial,
patients
received MIC before living donor kidney transplantation in addition to
post-transplant immunosuppression. Primary outcome measure was the
frequency of
adverse events (AE) until day 30 (study phase) with follow-up to day
360. MIC
infusions were extremely well tolerated. No 69 AE occurred which was
related to
MIC infusion. No donor-specific human leukocyte antigen antibodies or
rejection
episodes were noted even though the patients received donor mononuclear
cells
prior to transplantation. Patients with low immunosuppression during
follow-up
showed no in vitro reactivity against stimulatory donor blood
cells on
day 360 while reactivity against third party cells was preserved.
Frequencies
of CD19+CD24highCD38high transitional B lymphocytes (Breg) increased
from a
median of 6% before MIC infusion to 20% on day 180, which was 19- and
68-fold
higher, respectively, than in two independent cohorts of transplanted
controls.
The majority of Breg produced immunosuppressive cytokine IL-10.
MIC-treated
patients showed the Immune Tolerance Network operational tolerance
signature. In conclusion, MIC administration
was
safe and could be a future tool for the targeted induction of
tolerogenic Breg.
Morath et
al. (2020) J. Clin.
Invest. 26, 2364-2376.  |
The impact of
the secretome of activated pancreatic stellate cells on growth and
differentiation of pancreatic tumour cells
Pancreatic ductal
adenocarcinoma (PDAC) exists in a complex desmoplastic
microenvironment. As
part of it, pancreatic stellate cells (PSCs) provide a fibrotic niche,
stimulated by a dynamic communication between activated PSCs and tumour
cells. Investigating how PSCs contribute to tumour development
and for identifying
proteins that the cells secrete during cancer progression, we studied
by means
of complex antibody microarrays the secretome of activated PSCs. A
large number
of secretome proteins were associated with cancer-related functions,
such as
cell apoptosis, cellular growth, proliferation and metastasis.
..
Their effect on
tumour cells could be confirmed by growing tumour cells in medium
conditioned
with activated PSC secretome. Analyses of the tumour cells’ proteome
and mRNA
revealed a strong inhibition of tumour cell apoptosis, but promotion of
proliferation and migration.
..
Many cellular proteins that exhibited variations
were found to be under the regulatory control of eukaryotic translation
initiation factor 4E (eIF4E), whose expression was triggered in tumour
cells
grown in the secretome of activated PSCs. Inhibition by an eIF4E siRNA
blocked
the effect, inhibiting tumour cell growth in
vitro.
..
Our findings show that activated PSCs acquire a
pro-inflammatory
phenotype and secret proteins that stimulate pancreatic cancer growth
in an
eIF4E-dependent manner, providing further insight into the role of
stromal
cells in pancreatic carcinogenesis and cancer progression.
..
Marzoq et
al. (2019) Sci. Rep. 9,
5303. 
Figure legend: Scheme of the overall experimental set-up. First,
the protein content of the secretome of activated PSCs was analysed and
predictions were made about the functional consequences, which the
secreted proteins would have in recipient cells. Second, tumour cells
were grown in media conditioned with secretome. The intracellular
proteome was
studied and used for functional predictions. The predictions from
secretome and intracellular proteome were compared and validated by
investigating the actual functional variations observed and by
identifying
relevant regulative factors.
|
|
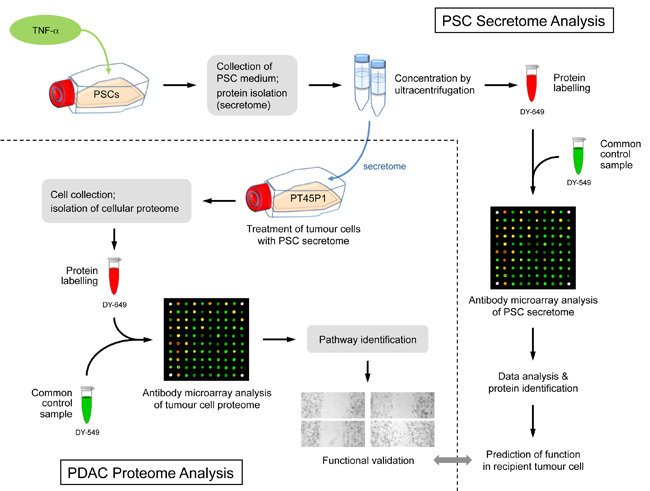
|
The structural basis of nanobody
unfolding reversibility and thermoresistance
..
|
Nanobodies represent
the variable binding domain of camelid heavy-chain antibodies and are
employed
in a rapidly growing range of applications in biotechnology and
biomedicine.
Their success is based on unique properties including their assumed
ability to reversibly
refold after denaturation. By characterizing nearly 70 nanobodies, we
show
that, opposed to common assumption, irreversible aggregation does occur
for
many binders upon heat denaturation, potentially affecting
application-relevant
parameters like stability, affinity and immunogenicity. However, by
deriving
aggregation propensities from apparent melting temperatures, we show
that an
optional disulfide bond suppresses nanobody aggregation. This effect is
further
enhanced by increasing the length of a complementarity determining loop
which,
although expected to destabilize, contributes to nanobody stability.
The effect
of such variations depends on environmental conditions, however.
Nanobodies
with two disulfide bonds, for example, are prone to lose their
functionality in
the cytosol. Our study suggests strategies to engineer nanobodies that
exhibit
optimal performance parameters and gives insights into general
mechanisms which
evolved to prevent protein aggregation.
|
|
|
Figure legend: Parameters determined in the nanobody
analysis. (A) A typical nanobody scaffold is shown. CDR
loops are
highlighted: CDR1, blue; CDR2, orange; CDR3, red. Hallmark positions
are shown
as black sticks, conserved and optional disulfide bonds as yellow
sticks. (B
& C) Parameters obtained from differential scanning flourimetry and
turbidity assays at a
temperature range of 25°C to 95°C. (B) Upper panel: The ratio
of intrinsic
protein fluorescence emission (350 nm/330 nm) reports about the onset
temperature of unfolding (Ton) and the melting point (Tm) during the
heating
phase. A difference of zero between initial and final ratio values
after a
complete temperature cycle (black arrow) would indicate complete
reversibility.
Lower panel: The turbidity trace of the heating phase yields the onset
temperature of aggregation (Ts)
and the turbidity integral (blue shaded area); the latter serves as a
qualitative measure of aggregation. If Ts occurs during the cooling
phase, the
turbidity integral is determined in reverse orientation. (C) Upper
panel:
Apparent melting temperature (Tm) values yield the ΔTm shift when
aggregation
is modulated by the nanobody concentration. The ΔTm shift can serve as
a
measure of aggregation propensity. Lower panel: the directly related
turbidity
traces are shown.
Kunz et al. (2019) Protein Eng. Des. Sel. 32,
gzz017.
Kunz et
al. (2018) Sci. Rep. 8,
7934. 
Kunz et al. (2015) BBA-Gen.
Subjects 1861,
2196-2205.
|
..
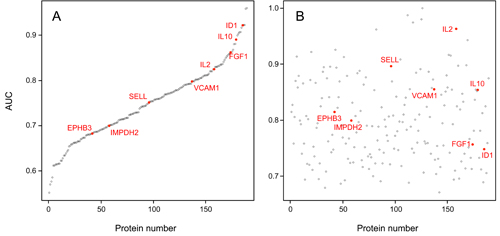
AUC values of the 189 individual serum markers. Analysis by Receiver Operating Characteristic (ROC)
curves was performed for all identified serum protein markers
individually.
Panel A shows the result calculated from the training set; the
respective AUC
values are shown, ranging from 55.2% to 96.0%. In panel B, the AUC
values are
shown as calculated for the individual marker molecules in the test
set. For
presentation, the order of the markers along the x-axis was kept as in
panel A,
highlighting the limited degree of reproducibility for individual
markers.
|
|
Comparison
of the tumour cell secretome and patient sera for an accurate
serum-based diagnosis of pancratic ductal adenocarcinoma
Pancreatic
cancer is the currently most lethal malignancy. Toward an accurate
diagnosis of
the disease in body liquids, we studied the protein composition of the
secretomes of 16 primary and established cell lines of pancreatic
ductal
adenocarcinoma (PDAC). Compared to the secretome of non-tumorous cells,
112
proteins exhibited significantly different abundances. Functionally,
the
proteins were associated with PDAC features, such as decreased
apoptosis,
better cell survival and immune cell regulation.
..
The result was
compared to
profiles obtained from 164 serum samples from two independent cohorts –
a
training and a test set – of patients with PDAC or chronic pancreatitis
and
healthy donors. Eight of the 112 secretome proteins exhibited similar
variations in their abundance in the serum profile specific for PDAC
patients,
which was composed of altogether 189 proteins.
..
The 8 markers shared by
secretome and serum yielded a 95.1% accuracy of distinguishing PDAC
from
healthy in a Receiver Operating Characteristic curve analysis, while
any number
of serum-only markers produced substantially less accurate results.
Utility of
the identified markers was confirmed by classical enzyme linked
immunosorbent
assays (ELISAs). The study highlights the value of cell secretome
analysis as a
means of defining reliable serum biomarkers.
..
..
Mustafa et
al. (2017) Oncotarget
8, 11963-11976. 
|
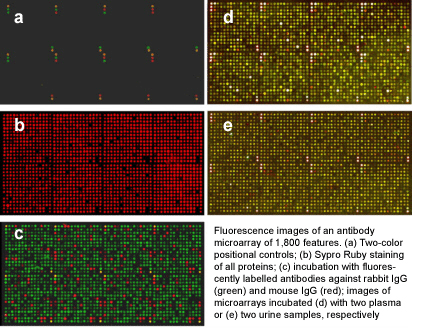
|
|
Detailed
protocols for expression profiling on antibody microarrays

As a
multiplexing technique, antibody
microarrays facilitate the highly parallel detection of thousands of
different
analytes from very small sample volumes of only few microliters. This
is
combined with a high sensitivity in the picomolar to femtomolar range,
which is
similar to the sensitivity of ELISA, the gold standard for protein
quantification.
In order to obtain such sensitivities in a robust and reproducible
manner for complex analytes, it is essential to use an optimised
experimental layout, sample handling, labelling and incubation as well
as defined data
processing steps.
Based on earlier
work, we
continuously developed the
processing of microarrays and protein samples. In the publications
listed
below, our antibody microarray protocols for
multiplexed
expression profiling studies are described in detail; they permit
the analysis of the
abundance of
very many proteins in plasma, urine, cell and tissue samples.
|
|
|
|
Schröder et al. (2010) Antibody
Engineering, Vol. 2,
SpringerVerlag, 429-445. |
 |
|
|
|
|
Schröder et al. (2010) Mol. Cell. Prot.
9, 1271-1280. |
 |
|
|
|
|
Alhamdani et al.
(2010) Proteomics 10, 3203-3207. |
 |
|
|
|
|
Schröder et al. (2011) Protein Micoarrays - Meth. Mol. Biol., Springer, 203-221.
|
|
|
|
|
|
|
|
|
|
|
|
|
.
|